Improved differential-neural cryptanalysis for round-reduced Simeck32/64
Deep learning has led to great improvements recently on a number of difficult tasks. In CRYPTO 2019, Gohr innovatively integrated deep learning with differential cryptanalysis, specifically applied to Speck32/64, resulting in developing a neural distinguisher that outperforms the DDT-based distinguisher. Applying differential neural cryptanalysis methods to more cryptographic algorithms is an issue worth studying. To solve […]

Deep learning has led to great improvements recently on a number of difficult tasks.
In CRYPTO 2019, Gohr innovatively integrated deep learning with differential cryptanalysis, specifically applied to Speck32/64, resulting in developing a neural distinguisher that outperforms the DDT-based distinguisher. Applying differential neural cryptanalysis methods to more cryptographic algorithms is an issue worth studying.
To solve the problems, a research team led by Liu ZHANG published their new research on 15 Dec 2023 in Frontiers of Computer Science co-published by Higher Education Press and Springer Nature.
The team used multiple convolutional layers with different kernel sizes based on the round function of Simeck32/64 to capture the characteristics of the ciphertext in multiple dimensions. Compared with existing research results, the accuracy and number of rounds of the differential-neural distinguisher for Simeck32/64 are improved.
In the research, they improve the Inception neural network according to the round function of Simeck32/64. To capture the connections between ciphertext pairs, they use multiple ciphertext pairs to form a sample as input to the neural network. These approaches enabled us to improve the accuracy of (9-12)-round differential-neural distinguisher (ND).
To establish solid baselines for ND, they compute the full distribution of differences induced by the input difference (0x0000, 0x0040) up to 13 rounds for Simeck32/64. To make a fair comparison with ND, they investigate the accuracy of DDT-distinguishers (DD) with multiple ciphertext pairs under independent assumptions. The comparison shows that the 9-, 10-round NDs achieve higher accuracy than the DD. This demonstrates that the ND contains more information than the DD.
Firstly, they found some (simultaneous-) neutral bit-sets for a 3-round differential. After comprehensive improvements in many aspects, they finally improve the 15-round and launch the first practical 16 and 17-round key recovery attacks for Simeck32/64 based on ND.
DOI: 10.1007/s11704-023-3261-z
Credit: Liu ZHANG, Jinyu LU, Zilong WANG, Chao LI
Deep learning has led to great improvements recently on a number of difficult tasks.
In CRYPTO 2019, Gohr innovatively integrated deep learning with differential cryptanalysis, specifically applied to Speck32/64, resulting in developing a neural distinguisher that outperforms the DDT-based distinguisher. Applying differential neural cryptanalysis methods to more cryptographic algorithms is an issue worth studying.
To solve the problems, a research team led by Liu ZHANG published their new research on 15 Dec 2023 in Frontiers of Computer Science co-published by Higher Education Press and Springer Nature.
The team used multiple convolutional layers with different kernel sizes based on the round function of Simeck32/64 to capture the characteristics of the ciphertext in multiple dimensions. Compared with existing research results, the accuracy and number of rounds of the differential-neural distinguisher for Simeck32/64 are improved.
In the research, they improve the Inception neural network according to the round function of Simeck32/64. To capture the connections between ciphertext pairs, they use multiple ciphertext pairs to form a sample as input to the neural network. These approaches enabled us to improve the accuracy of (9-12)-round differential-neural distinguisher (ND).
To establish solid baselines for ND, they compute the full distribution of differences induced by the input difference (0x0000, 0x0040) up to 13 rounds for Simeck32/64. To make a fair comparison with ND, they investigate the accuracy of DDT-distinguishers (DD) with multiple ciphertext pairs under independent assumptions. The comparison shows that the 9-, 10-round NDs achieve higher accuracy than the DD. This demonstrates that the ND contains more information than the DD.
Firstly, they found some (simultaneous-) neutral bit-sets for a 3-round differential. After comprehensive improvements in many aspects, they finally improve the 15-round and launch the first practical 16 and 17-round key recovery attacks for Simeck32/64 based on ND.
DOI: 10.1007/s11704-023-3261-z
Journal
Frontiers of Computer Science
DOI
10.1007/s11704-023-3261-z
Method of Research
Experimental study
Subject of Research
Not applicable
Article Title
Improved differential-neural cryptanalysis for round-reduced SIMECK32/64
Article Publication Date
2-Dec-2023
What's Your Reaction?
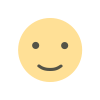
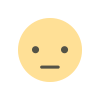
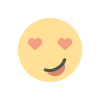
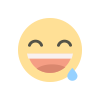
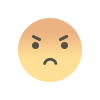
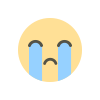
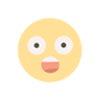