Image Selection Impact on Lung Cancer Radiomics
In the rapidly evolving field of medical imaging and artificial intelligence, the extraction of radiomics features from lung cancer images stands as a pivotal step toward enhancing diagnosis, prognosis, and personalized treatment strategies. A recent study published in BMC Cancer dives deeply into this domain, offering groundbreaking insights into how the selection of imaging parameters […]

In the rapidly evolving field of medical imaging and artificial intelligence, the extraction of radiomics features from lung cancer images stands as a pivotal step toward enhancing diagnosis, prognosis, and personalized treatment strategies. A recent study published in BMC Cancer dives deeply into this domain, offering groundbreaking insights into how the selection of imaging parameters and segmentation methodologies influence the robustness and reliability of lung cancer radiomics features, particularly using the popular open-source 3D-Slicer software.
The investigation addresses a crucial ambiguity lingering in clinical radiomics practice: whether variations in image acquisition and segmentation approaches materially affect the extraction of meaningful radiomics data. Lung cancer imaging, especially for non-small cell lung carcinoma (NSCLC), frequently leverages CT scans that can be captured in different windowing settings—namely lung window and mediastinal window—and with or without intravenous contrast enhancement. Each of these factors potentially alters tumor visualization and subsequently, the radiomics signatures derived.
To systematically examine these variables, the authors conducted a methodical study using images from 22 lung cancer patients sourced from The Cancer Imaging Archive (TCIA). The cohort balanced adenocarcinoma and squamous cell carcinoma cases equally, providing a robust dataset for comparative analyses. Tumor segmentation was performed on both lung window and mediastinal window images for plain (non-contrast) and enhanced scans. Manual delineation was employed for lung window images, while the mediastinal window leveraged an automatic segmentation protocol followed by manual corrections to ensure accuracy.
The extracted radiomics features numbered 107 per image set, totaling 88 sets overall. These features spanned various categories, including intensity-based metrics, shape descriptors, and texture quantifications that collectively offer a comprehensive characterization of tumor heterogeneity and morphology. Statistical analyses employed included normality tests (Shapiro-Wilk), variance analysis (ANOVA), and non-parametric Friedman tests to discern significant differences across groups. The study ventured beyond mere feature extraction, probing which imaging parameters might distinctly influence the radiomic profiles.
Results showcased that among the vast array of features extracted, 33 demonstrated significant variability contingent upon image selection and segmentation methodology. Particularly, comparisons between different window settings and contrast phases revealed nuanced yet critical differences. For instance, enhanced lung window images produced two features significantly divergent from plain scans, suggesting limited but notable influence of contrast injection under lung window conditions. Contrastingly, features extracted from different windows—especially between enhanced lung and plain mediastinal windows—exhibited far more pronounced discrepancies, with as many as twelve features differing significantly.
Moreover, when comparing plain scans to enhanced mediastinal window images, only one feature showed a significant shift, highlighting that enhancement may have a more subdued impact within certain imaging windows. Distinct comparisons between the lung and mediastinal windows across both plain and enhanced scans unveiled 13 to 14 features with significant differences, underscoring that the choice of window significantly affects feature representation.
Perhaps most compellingly, the study’s pathological subgroup analysis illuminated that radiomics features could differentiate squamous cell carcinoma from adenocarcinoma with substantial significance, uncovering 54 features that reliably varied between these major NSCLC subtypes. This finding signals the tremendous potential of radiomics to non-invasively classify tumor histology, augmenting pathological diagnostics with quantitative imaging biomarkers.
Fundamentally, the research emphasizes that while contrast enhancement imparts some changes to radiomics features, the selection of imaging window for segmentation exerts a much greater influence. This has profound implications for standardizing radiomics workflows, as ensuring consistency in segmentation windows could dramatically improve feature reproducibility and reliability for longitudinal studies or multi-center trials.
The reliance on 3D-Slicer for segmentation and radiomics extraction further grounds the study’s conclusions in accessible, open-source tools that are increasingly popular among clinicians and researchers alike. However, the study also reflects on the delicate balance between manual and automatic segmentation techniques. The hybrid approach adopted—manual segmentation in lung windows and semi-automated segmentation with manual adjustments in mediastinal windows—highlights practical challenges in maintaining quality and reproducibility.
This research not only paves the way for more standardized radiomics pipelines but also alerts the scientific community to critical methodological considerations that could otherwise confound study results. The significant sensitivity of features to window setting suggests that future multicenter studies should strictly define and adhere to segmentation protocols to ensure replicability.
Furthermore, the promising discrimination between adenocarcinoma and squamous cell carcinoma by imaging features lays a foundation for subsequent studies focused on radiogenomics and integrating molecular profiles with imaging data. Such integrative approaches may ultimately facilitate tailored therapeutic regimens, sparing patients from invasive biopsies when possible.
In conclusion, the study by Liu and colleagues reinforces the concept that image acquisition and segmentation decisions are not mere technicalities but integral components shaping the radiomics landscape. The insight that the window chosen for image segmentation profoundly influences feature extraction urges a reevaluation of current radiomics practices. By adopting standardized protocols and recognizing the nuanced effects of image parameters, the oncology community can harness radiomics more effectively to improve patient outcomes.
This pioneering work bridges the gap between image processing technicalities and clinical applicability, highlighting that the devil truly lies in the details when it comes to radiomics. With radiomics poised to revolutionize lung cancer management, such meticulous investigations serve as essential guides steering this promising technology from research to real-world impact.
The findings call upon researchers, clinicians, and software developers to collaborate in crafting unified frameworks that streamline radiomics feature extraction, ensuring robustness and clinical relevance. As the oncology field embraces precision medicine, leveraging imaging data to its fullest potential will demand adherence to validated protocols and awareness of methodological variabilities.
Ultimately, this study invites an exciting horizon where radiomics, supported by standardized segmentation and imaging strategies, could transform how lung cancers are detected, characterized, and treated — bringing personalized oncology closer to reality through the power of data-driven imaging science.
Subject of Research: The impact of image selection and segmentation parameters on the extraction of lung cancer radiomics features using 3D-Slicer software.
Article Title: The influence of image selection and segmentation on the extraction of lung cancer imaging radiomics features using 3D-Slicer software
Article References:
Liu, C., He, Y. & Luo, J. The influence of image selection and segmentation on the extraction of lung cancer imaging radiomics features using 3D-Slicer software. BMC Cancer 25, 728 (2025). https://doi.org/10.1186/s12885-025-14094-z
Image Credits: Scienmag.com
DOI: https://doi.org/10.1186/s12885-025-14094-z
Tags: 3D-Slicer software for medical imagingadenocarcinoma vs squamous cell carcinomaCancer Imaging Archive datasetclinical implications of radiomics datacontrast enhancement effects on imagingCT scan parameters for lung cancerimage selection impact on radiomicslung cancer radiomicsnon-small cell lung carcinoma imagingrobustness of radiomics featuressegmentation methodologies in radiomicstumor visualization in lung cancer
What's Your Reaction?
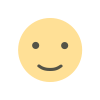
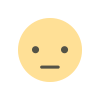
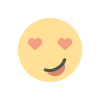
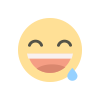
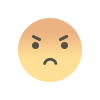
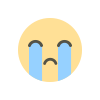
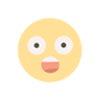