FIFAWC: Enhanced Dataset Delivers In-Depth Annotations and Semantics for Advancing Group Activity Recognition
Revolutionizing Group Activity Recognition with FIFAWC: A New Dataset Unveiled In the fast-evolving world of computer vision, the need for comprehensive data to train and enhance machine learning models has never been more essential. Recent advancements in artificial intelligence have opened new doors in various areas, with Group Activity Recognition (GAR) emerging as a particularly […]

Revolutionizing Group Activity Recognition with FIFAWC: A New Dataset Unveiled
In the fast-evolving world of computer vision, the need for comprehensive data to train and enhance machine learning models has never been more essential. Recent advancements in artificial intelligence have opened new doors in various areas, with Group Activity Recognition (GAR) emerging as a particularly promising field. This area focuses on understanding collective actions in videos, a task that resonates with real-world scenarios more than isolated actions. However, the existing datasets utilized in GAR research have critical limitations that hinder progress. Traditional approaches often rely on single annotations for a specific group activity per sample, which does not reflect the complexity of real-life videos that typically showcase multiple concurrent activities.
The necessity for a more nuanced approach called for innovation in data collection and annotation strategies. Researchers are beginning to recognize that to achieve significant breakthroughs in GAR, datasets must encompass not only multiple group activity instances but also rich semantic information that accurately conveys the context of each action. Addressing these concerns, a research team led by Wang Yun-Hong from Beihang University has taken a monumental step forward with their newly published dataset, FIFAWC. This novel dataset is poised to transform the landscape of Group Activity Recognition by providing a more authentic representation of group activities in video footage.
At the core of FIFAWC’s contributions is its comprehensive annotation approach. Unlike existing datasets that focus predominantly on a singular group activity within a sample, FIFAWC takes the bold leap of thoroughly annotating all group activities present in each video segment. This meticulous attention to detail not only enhances the complexity of the dataset but also aligns it more closely with real-world scenarios, providing researchers with a fertile ground for more advanced experimentation and development.
Moreover, FIFAWC stands out through its provision of semantic descriptions accompanying each video clip. These annotations come from professional sports commentators, ensuring a level of accuracy and professionalism that has been sorely lacking in previous datasets. By embedding rich semantic information into the dataset, FIFAWC presents itself as a versatile data resource, beneficial not only for group activity recognition but also for related endeavors such as video captioning and retrieval tasks. This development is a game-changer, as it addresses the pressing need for datasets that make it easier to train algorithms capable of understanding nuanced human behavior in group settings.
Additionally, FIFAWC introduces an entirely new scenario for group activity recognition research: soccer match footage. This choice of context adds layers of complexity that have not been seen in prior datasets. Soccer matches are characterized by expansive spatial dynamics and rapid movements, providing a unique challenge for researchers looking to make significant advancements in their methodologies. The intricate nature of soccer, including dynamic camera movements and the presence of smaller targets within the frame, reflects the unpredictable scenarios that real-world recognition systems must contend with.
As the research team engaged in benchmarking FIFAWC, they executed evaluations across two specific tasks: traditional group activity recognition and innovative group activity video captioning. The results from their experiments were illuminating. In traditional GAR assessments, they utilized both a classical detector-based approach, ARG, as well as a cutting-edge detector-free methodology, DFWSGAR. The findings underscored a notable disparity; while high accuracy was observed at the category level, the sample-level accuracy revealed considerable challenges due to the inherent complexity of having multiple group activities in each sample.
Turning the spotlight towards video captioning, the researchers employed the conventional captioning method, PDVC, alongside the more revolutionary Large Language Model-based approach, VTimeLLM. The comparison shed light on the inadequacies of existing methodologies when applied to FIFAWC. While PDVC achieved commendable performance attribution on the ActivityNet dataset, its results on FIFAWC highlighted the urgent need for further refinement in captioning techniques tailored for group activities. This disparity serves as a clarion call for the research community, urging them towards innovation and adaptation in their approaches.
The implications of FIFAWC’s release are profound. As research teams grapple with the intricacies involved in group activity recognition, they are equipped with a dataset that mirrors the multifaceted nature of human interaction. With its comprehensive annotations, professional commentaries, and focus on dynamic sports footage, FIFAWC represents a step-change in the availability of data for researchers striving to push the boundaries of computer vision.
As we witness this groundbreaking shift, it is essential for the academic and professional communities to rally around the promising opportunities that FIFAWC brings to the table. With the increased depth and variety of data on offer, the time has come to foster collaborations that leverage this dataset for advancing technologies in video surveillance, autonomous driving, and various other applications where understanding collective human behavior is critical. The bold strides made by Wang Yun-Hong and his team at Beihang University signal a new dawn for group activity recognition research.
As we look forward, it will be intriguing to see how this dataset shapes future methodologies and inspires novel algorithms that can discern complex activities in real-time video streams. The challenges posed by FIFAWC may be substantial, but they also serve as stepping stones towards achieving the sophisticated understanding of group dynamics that practitioners in artificial intelligence envision. The road ahead is challenging but bright, and it will be exciting to follow the developments in this vibrant field.
In conclusion, the introduction of FIFAWC stands as a testament to researcher ingenuity and the unwavering pursuit of knowledge in the realm of computer vision. With FIFAWC now at their disposal, researchers can delve deeper into group activity recognition, paving the way for smarter systems capable of interpreting the richly woven tapestry of human actions. As such, FIFAWC is more than just a dataset; it is a vital catalyst for the evolution of artificial intelligence applications aimed at understanding human behavior in all its myriad forms.
Subject of Research:
Article Title: FIFAWC: A Dataset with Detailed Annotation and Rich Semantics for Group Activity Recognition
News Publication Date: 15-Dec-2024
Web References: DOI
References: To be updated.
Image Credits: Duoxuan PEI, Di HUANG, Yunhong WANG
Keywords
Computer vision, Group Activity Recognition, Dataset, Video captioning, Semantic annotation, AI, Machine learning, Complex interactions.
What's Your Reaction?
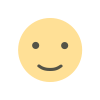
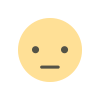
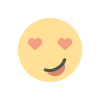
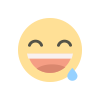
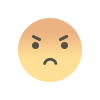
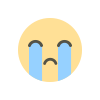
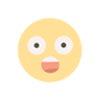