Exploring Federated Transfer Learning: Key Challenges, Innovative Methods, and Diverse Applications
Federated Learning (FL) has emerged as a leading-edge distributed machine learning approach that not only enhances performance in model training but also prioritizes data privacy. As organizations increasingly embrace this paradigm, it is crucial to address the various challenges inherent in traditional Federated Learning methods. These challenges primarily arise from data heterogeneity, system variability, and […]

Federated Learning (FL) has emerged as a leading-edge distributed machine learning approach that not only enhances performance in model training but also prioritizes data privacy. As organizations increasingly embrace this paradigm, it is crucial to address the various challenges inherent in traditional Federated Learning methods. These challenges primarily arise from data heterogeneity, system variability, and the limited availability of labeled data. Researchers have recognized the need for innovative solutions and have turned their attention to Federated Transfer Learning (FTL), which merges the concepts of Transfer Learning with Federated Learning. This synthesis aims to overcome the specific limitations that FL encounters while concurrently enabling collaborative learning across decentralized datasets.
On December 15, 2024, an influential research team led by Fuzhen Zhuang published a highly relevant study in the journal Frontiers of Computer Science. This publication provides a deep dive into the multifaceted realm of Federated Transfer Learning, meticulously examining its potential to revolutionize the landscape of distributed machine learning. The significance of this research lies not only in its systematic classification of FTL scenarios but also in its comprehensive overview of existing methodologies addressing these unique challenges. The work serves as a springboard for both researchers and practitioners operating within the intersecting fields of machine learning, data privacy, and distributed computing.
The research categorizes the diverse settings of FTL into six primary classifications: homogeneous FTL, heterogeneous FTL, dynamic heterogeneous FTL, model adaptive FTL, semi-supervised FTL, and unsupervised FTL. Each category represents a distinct approach to overcoming the challenges posed by Federated Learning’s framework. For instance, homogeneous FTL deals with scenarios where participant datasets share similar characteristics, while heterogeneous FTL focuses on managing data that exhibits significant variability among participants. The dynamic variant addresses scenarios where data may evolve over time, highlighting the need for adaptable learning strategies.
As researchers navigate these categories, they also emphasize the practical significance of model design specific to FTL environments. The essence of this approach lies in its ability to adapt models that can not only learn from local datasets but can also integrate knowledge gleaned from other participants during collaborative training sessions. This paradigm aims to enhance predictive performance while maintaining strict adherence to privacy regulations, ensuring that sensitive information remains within local domains.
Privacy-preserving mechanisms form a critical component of the discussion surrounding FTL. The ability to exchange knowledge without compromising individual data privacy is paramount in ensuring the acceptance and viability of Federated Transfer Learning across diverse industries. The authors systematically summarize various algorithms that underpin FTL methodologies, highlighting their effectiveness in managing trade-offs between collaboration and privacy. The future of FTL hinges on developing smarter algorithms that can facilitate knowledge sharing while maintaining robust privacy standards.
The survey further outlines innovative communication architectures crucial to the efficient implementation of FTL. Effective communication among participating entities is fundamental to achieving the desired model convergence in a Federated Learning scenario. The study discusses the intricacies of different communication protocols and their impact on the speed and efficiency of learning processes, emphasizing that improved communication strategies are paramount for driving advancements in FTL. Such analyses resonate with organizations looking to implement Federated Learning solutions in real-world applications.
Moreover, the survey explores the various domains where Federated Transfer Learning can be applied. From healthcare, where patient data privacy is a primary concern, to financial services, where regulatory compliance is paramount, the potential applications of FTL are vast. The authors underline the importance of establishing robust systems and infrastructures that support the successful implementation of FTL frameworks. By detailing these applications, the work effectively showcases the practical relevance of Federated Transfer Learning in addressing pressing industry challenges.
Looking towards the future, the authors articulate a vision for the continued evolution and adoption of Federated Transfer Learning. They encourage researchers to delve deeper into the underlying mechanisms that could enhance FTL’s applicability, particularly in settings characterized by extreme data disparity or limited labeled datasets. There is an implicit call for collaborative research efforts aimed at refining FTL methodologies to not only improve performance but also facilitate broader deployment across diverse sectors.
The comprehensive nature of this survey positions it as an invaluable resource for both new entrants and seasoned experts in the sphere of machine learning. By encapsulating theoretical viewpoints alongside practical applications, the authors provide a holistic perspective on the current state of Federated Transfer Learning. This foundational understanding is essential for driving future research initiatives and bridging the gap between theory and practice in data privacy-oriented environments.
In summary, the publication delineates a significant landmark in the blossoming field of Federated Transfer Learning. By providing clarity on its underlying principles, categorizing its diverse settings, and showcasing its potential applications, the study strengthens the foundation upon which further innovation can be built. As the boundaries of distributed machine learning continue to expand, research efforts such as this will inevitably shape the landscape, paving the way for transformative advancements that prioritize both performance and privacy in the age of digital data.
Subject of Research: Federated Transfer Learning
Article Title: A comprehensive survey of federated transfer learning: challenges, methods and applications
News Publication Date: 15-Dec-2024
Web References: N/A
References: N/A
Image Credits: Wei GUO, Fuzhen ZHUANG, Xiao ZHANG, Yiqi TONG, Jin DONG
Keywords: Federated Learning, Federated Transfer Learning, data privacy, distributed machine learning, Transfer Learning, privacy-preserving mechanisms, communication architectures.
What's Your Reaction?
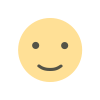
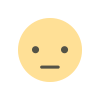
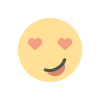
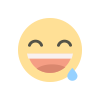
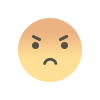
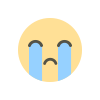
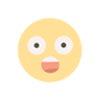