Estimating Inbreeding and Relatedness via 9 IBD Coefficients
In the ever-evolving field of genetics, accurate estimation of identity by descent (IBD) coefficients remains a cornerstone for understanding the underlying relatedness and inbreeding within populations. A recent breakthrough has emerged with the release of EMIBD9, an innovative computer program designed to delve deeper into the genetic relationships between individuals through precise computation of the […]

In the ever-evolving field of genetics, accurate estimation of identity by descent (IBD) coefficients remains a cornerstone for understanding the underlying relatedness and inbreeding within populations. A recent breakthrough has emerged with the release of EMIBD9, an innovative computer program designed to delve deeper into the genetic relationships between individuals through precise computation of the nine condensed IBD coefficients, collectively denoted as Δ = {Δ₁, Δ₂, …, Δ₉}. These coefficients encapsulate the probabilities that two individuals share zero to four alleles identical by descent across loci, offering unparalleled insight into their genetic kinship and inbreeding levels.
Traditional methods for estimating IBD coefficients often grapple with limitations when applied to samples containing close relatives or populations exhibiting inbreeding. This challenge stems from the reliance on accurate allele frequency estimates, which are frequently inferred under assumptions of large, unrelated, and non-inbred samples. Recognizing these constraints, EMIBD9 introduces two distinct likelihood-based approaches that cater separately to small, complex samples and large, predominantly unrelated datasets. At its core, the program navigates the delicate balance between computational feasibility and inferential accuracy, ensuring robust estimations under varying research conditions.
The first method implemented in EMIBD9 harnesses the power of the Expectation-Maximization (EM) algorithm, renowned for its iterative refinement capabilities in the presence of missing or latent data variables. This approach is particularly adept at managing scenarios where sample sizes are limited or enriched in close relatives, conditions under which allele frequencies are challenging to estimate directly. By jointly estimating the condensed IBD coefficients and the underlying allele frequencies in an iterative fashion, the algorithm self-corrects, incrementally improving both sets of parameters with each iteration. This joint estimation stands as a marked departure from standard practices and mitigates biases introduced by inaccurate frequency assumptions.
Conversely, the second method caters to large-scale genetic data where the assumption of a predominantly unrelated, non-inbred sample holds true. Eschewing the computational demands of iterative frequency updates, this approach expedites the process by estimating only the condensed IBD coefficients. While this method may sacrifice some nuance in allele frequency accounting, its speed and efficiency make it invaluable for genome-wide association studies or population genomic surveys encompassing thousands of individuals. The dual-method framework embodied in EMIBD9 underscores the flexibility necessary for modern genetic research, seamlessly adapting to diverse sampling strategies.
Beyond the mathematical intricacies underpinning EMIBD9, its cross-platform availability significantly broadens accessibility for researchers worldwide. Compatible across Windows, Mac, and Linux operating systems, the program facilitates integration into existing computational pipelines irrespective of user preferences or institutional constraints. Notably, the Windows edition boasts a Graphical User Interface (GUI) designed to streamline data input and enhance result visualization, an asset particularly appreciated by researchers who may lack extensive bioinformatics training. This blend of computational power and user-friendly design promotes wider adoption of advanced IBD estimation methods across genetics laboratories.
One of the program’s salient features lies in its capacity to simulate genotype data via the GUI. This simulation tool transcends mere data generation; it operates as a critical investigative instrument for dissecting the factors influencing relatedness estimation accuracy. Through simulated datasets, users can evaluate how sample composition, marker density, allele frequency distributions, and inbreeding levels interact to impact the fidelity of relatedness inference. Such experiments allow researchers to optimize experimental designs proactively, tailoring marker panels and sampling schemes before committing valuable resources to genotyping efforts.
Delving into the mathematical foundation, the nine condensed IBD coefficients embodied in Δ encompass all possible identity states of two diploid individuals at a locus. These coefficients quantify the probability of sharing zero, one, or two alleles identical by descent from common ancestors, synthesizing complex genealogical information into concise parameters. From these coefficients, downstream calculations of relatedness or kinship coefficients and inbreeding levels become feasible, supporting varied applications from pedigree reconstruction and forensic analyses to conservation genetics.
Incorporating these coefficients into likelihood-based frameworks addresses the probabilistic nature of genotype data and genotypic uncertainty. Unlike simplistic identity-by-state metrics, which may confound allele sharing due to population structure or allele frequency variations, likelihood approaches incorporate allele frequency information directly, enhancing specificity. The EM algorithm’s role in simultaneously refining these frequencies alongside relatedness coefficients represents a methodological innovation with broad implications for accuracy and bias reduction.
The impact of EMIBD9 extends beyond theoretical statistics, promising tangible benefits in numerous applied genetics realms. For instance, in natural populations where inbreeding and kinship patterns underlie evolutionary processes and conservation status, precise estimation of IBD parameters informs management decisions. By furnishing a robust toolset adaptable to varying sample characteristics, EMIBD9 empowers population geneticists to work confidently with datasets that previously posed analytical challenges.
Importantly, EMIBD9’s capacity to accommodate samples rich in close relatives addresses a significant gap in existing software. High relatedness within samples can distort allele frequency estimates if unaccounted for, cascading into inaccurate relatedness inferences. The joint estimation procedure alleviates this dependence on external allele frequency references, a boon particularly valuable in species lacking extensive genomic resources or populations subjected to non-random mating systems.
While EMIBD9’s advanced methodology represents a step forward, it also highlights ongoing challenges in genetic relatedness estimation. For example, distinguishing between different pedigree relationships with similar expected IBD sharing can remain elusive even with nine coefficients, especially when marker density or quality is limiting. Nonetheless, EMIBD9’s design facilitates incorporation of dense marker data and multi-locus information, which collectively improve discrimination power, fortifying its utility in comprehensive genetic studies.
The graphical visualization tools embedded in the program further democratize its capabilities, portraying complex IBD coefficient estimates in intuitive formats. Such visual summaries assist researchers in interpreting results, detecting anomalies, and communicating findings to broader audiences including stakeholders in breeding programs, conservation, and medical genetics. This educational facet enhances transparency and fosters confidence in interpretations derived from genetic data.
From a computational standpoint, EMIBD9 exemplifies efficient software engineering by integrating iterative algorithms with optimized likelihood calculations. Balancing the computational demands of EM steps with the needs for rapid analysis, particularly in large-scale datasets, required careful algorithmic refinement and testing. The inclusion of two tailored methods within a single platform reflects a pragmatic recognition of real-world research diversity, positioning EMIBD9 as a versatile and future-proof tool.
Looking ahead, the principles embedded in EMIBD9’s framework may inspire extensions encompassing additional genetic complexities such as linkage disequilibrium, haplotype structures, or polyploidy. Moreover, its modular architecture paves the way for integration with other genomic analysis pipelines, potentially synergizing with machine learning techniques to enhance inference robustness. These avenues suggest that EMIBD9 is not merely a static tool but a foundation for continued innovation in genetic relatedness estimation.
In conclusion, EMIBD9 signifies a meaningful stride in the quantitative analysis of genetic relatedness, addressing critical gaps by implementing dual likelihood-based methods suited for contrasting sample scenarios. Its incorporation of the EM algorithm to jointly estimate allele frequencies and IBD coefficients in challenging samples stands as a methodological landmark, while its efficiency-oriented alternative caters to large-scale data contexts. Available across major computing platforms with user-friendly interfaces, EMIBD9 democratizes access to sophisticated genetic analyses, promoting accurate and insightful inferences of relatedness and inbreeding that underpin foundational questions in genetics.
Subject of Research: Genetic relatedness estimation through condensed identity by descent (IBD) coefficients, inbreeding, and kinship analysis using genotype data.
Article Title: EMIBD9: Estimating 9 condensed IBD coefficients, inbreeding and relatedness from marker genotypes.
Article References:
Wang, J. EMIBD9: Estimating 9 condensed IBD coefficients, inbreeding and relatedness from marker genotypes. Heredity 134, 155–161 (2025). https://doi.org/10.1038/s41437-024-00739-5
Image Credits: AI Generated
DOI: April 2025
Tags: allele frequency estimation challengescomputational genetics advancementsEMIBD9 software applicationgenetic relatedness analysisIBD coefficient estimationidentity by descent calculationsinbreeding assessment methodsiterative refinement algorithms in geneticskinship and genetic relationshipslikelihood-based statistical approachespopulation genetics research techniquessmall sample genetic studies
What's Your Reaction?
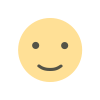
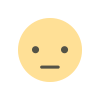
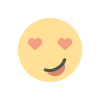
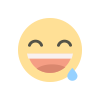
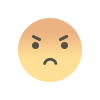
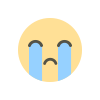
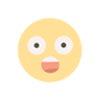