Dynamic Early Warning Model Predicts Flash Floods
In an era marked by escalating climate volatility, the threat of flash floods has become a critical concern for communities worldwide. These rapid-onset hydrological disasters strike with little warning, often causing devastating consequences for lives, infrastructure, and economies. Addressing this urgent challenge, a groundbreaking study published in the International Journal of Disaster Risk Science introduces […]

In an era marked by escalating climate volatility, the threat of flash floods has become a critical concern for communities worldwide. These rapid-onset hydrological disasters strike with little warning, often causing devastating consequences for lives, infrastructure, and economies. Addressing this urgent challenge, a groundbreaking study published in the International Journal of Disaster Risk Science introduces a pioneering early warning system that leverages advanced rainfall pattern identification to predict flash floods dynamically. Spearheaded by Yuan, W., Jing, B., Xu, H., and colleagues, this research has the potential to revolutionize how societies prepare for and respond to these perilous events.
Flash floods defy traditional warning methods due to their sudden nature and the localized characteristics of rainfall that lead to their formation. Conventional systems often rely on fixed threshold triggers, such as accumulated rainfall or river discharge levels, which may not fully capture the complexity of underlying meteorological processes. The new model proposed by Yuan et al. abandons rigid metrics in favor of a flexible, data-driven approach that analyzes rainfall patterns in real-time, allowing for adaptive forecasting attuned to evolving conditions. This dynamic early warning model integrates hydrological data with sophisticated pattern recognition algorithms, paving the way for faster and more accurate flood alerts.
Central to the model’s innovation is its deployment of machine learning techniques that scrutinize spatiotemporal rainfall distributions. By training on diverse meteorological data sets, the system learns to discern subtle yet significant variations in precipitation intensity, duration, and distribution that often precede flash flood events. Unlike traditional models, which might overlook atypical or nonlinear rainfall behaviors, this approach adapts continuously, refining its predictive capability as more data becomes available. This adaptability enables detection of complex precursors to flooding that human operators or static models cannot easily identify.
The research team emphasized that accurately characterizing rainfall patterns is essential because flash floods often result from localized torrents rather than widespread precipitation. Through high-resolution rainfall monitoring combined with real-time hydrological modeling, the system captures micro-scale phenomena such as storm cells and convective bursts. These meteorological features can generate extreme runoff within minutes, overwhelming drainage systems and river catchments. By identifying these intense, short-lived rainfall episodes through pattern recognition frameworks, the model anticipates flash flood triggers before conventional hydrological signs develop.
Notably, the model’s architecture is designed for scalability and integration with existing meteorological infrastructure. Accessibility and ease of deployment were prioritized, allowing municipal authorities and emergency services to incorporate the early warning system into their operational workflows rapidly. The software interfaces seamlessly with radar and satellite precipitation measurements, enabling continuous data ingestion. This system-level integration ensures that flood risk assessments can be continuously updated and disseminated, enhancing situational awareness among stakeholders.
Beyond algorithmic sophistication, the researchers rigorously validated the model using historical flash flood cases across varied geographic and climatic contexts. Retrospective analyses demonstrated that the system consistently outperformed baseline models, significantly reducing false alarms and missed events. Particularly in regions prone to sudden, intense rainfall—such as mountainous terrains and urbanized watersheds—the model’s sensitivity to critical rainfall signatures translated to ample lead time for warnings. This empirical validation underscores the model’s robustness and practical utility for real-world disaster risk reduction.
In addition to improving detection accuracy, the study highlights the crucial role of dynamic thresholds that adjust according to evolving weather conditions and antecedent soil moisture status. Flash flood susceptibility is often amplified when soils are saturated from prior rains, diminishing infiltration capacity and accelerating runoff. By incorporating real-time hydrological state variables into the forecasting process, the model fine-tunes its alert criteria, preventing either over-warning during benign conditions or delayed notices during critical vulnerabilities. This nuanced calibration marks a significant departure from static, one-size-fits-all thresholds commonly employed.
Equally important to the authors was the transparency of the algorithmic decision-making process. In disaster risk management, trust and interpretability are paramount for end-users who must make rapid, consequential choices. Therefore, the model incorporates interpretable machine learning modules that provide explanations for flood warnings, such as spotlighting key rainfall features that triggered the alert. This insight fosters user confidence and facilitates collaborative decision-making between technical staff, emergency responders, and policymakers, ultimately improving response effectiveness.
The deployment prospects of this early warning system extend beyond national borders, particularly as global climate patterns shift and extreme rainfall events become increasingly frequent and unpredictable. The adaptability of the rainfall pattern identification framework ensures its applicability across diverse hydrometeorological regimes. Regional calibration can tune the model’s parameters to reflect local climatology and terrain effects. Consequently, this approach presents a scalable solution to a universal hazard, equipping vulnerable regions worldwide with enhanced predictive tools to counter flash floods.
While the study marks a significant breakthrough, the authors also acknowledge challenges ahead, particularly regarding data availability and quality in resource-limited settings. Operationalizing the model depends on access to comprehensive meteorological measurements, including radar and satellite data, which may be scarce in some developing regions. The team advocates for international collaboration and investment in sustainable data collection networks to democratize access to advanced early warning technologies. Only through such integrated efforts can the benefits of this cutting-edge model be fully realized globally.
Moreover, the research prompts a re-examination of conventional flood mitigation strategies, encouraging a shift from reactive disaster response to proactive risk management. Early and accurate warnings enable communities to mobilize evacuations, secure infrastructure, and implement tailored contingency plans. The dynamic nature of the model allows adaptation to changing environmental conditions brought on by urban expansion, deforestation, or climate change, ensuring that flood preparedness keeps pace with evolving hazards. Thus, the innovation represents a cornerstone in building resilient cities and safeguarding vulnerable populations.
This work also opens avenues for further interdisciplinary research, merging hydrology, meteorology, data science, and emergency management. Future enhancements could involve integrating social vulnerability indices and infrastructure resilience data to produce comprehensive risk maps that guide targeted interventions. Additionally, coupling the rainfall pattern-based warnings with crowd-sourced flood observations and Internet-of-Things sensors could refine situational awareness down to neighborhood scales. Such multi-layered data fusion would empower authorities to deliver precision warnings and optimize resource allocation during flood emergencies.
From a technological standpoint, the study exemplifies the transformative potential of artificial intelligence in natural hazard forecasting. The use of dynamic, self-learning systems capable of decoding complex environmental signals heralds a paradigm shift away from deterministic models toward adaptive intelligence frameworks. This paradigm not only advances scientific understanding but also translates directly into lives saved and damages reduced. As extreme weather phenomena intensify worldwide, these innovations become indispensable tools in humanity’s efforts to coexist sustainably with nature’s forces.
In conclusion, the early warning model devised by Yuan, Jing, Xu, and their colleagues stands as a landmark achievement in flash flood prediction. By harnessing real-time rainfall pattern identification through adaptive machine learning, the system delivers unprecedented forecasting precision and timeliness. Its validated performance across diverse scenarios highlights its readiness for operational deployment, promising to enhance disaster risk mitigation globally. As climate change continues to challenge traditional preparedness paradigms, such dynamic early warning frameworks will be critical in safeguarding communities from the sudden fury of flash floods, potentially transforming hazard management in the decades to come.
Article Title: A Dynamic Early Warning Model for Flash Floods Based on Rainfall Pattern Identification
Article References:
Yuan, W., Jing, B., Xu, H. et al. A Dynamic Early Warning Model for Flash Floods Based on Rainfall Pattern Identification. Int J Disaster Risk Sci 15, 769–788 (2024). https://doi.org/10.1007/s13753-024-00593-3
Image Credits: AI Generated
Tags: adaptive forecasting techniquesadvanced pattern recognition algorithmsclimate volatility impactcommunity preparedness for floodsdynamic early warning systememergency response to flash floodsflash flood predictionhydrological disasters managementinfrastructure resilience to floodingInternational Journal of Disaster Risk Sciencerainfall pattern identificationreal-time data analysis
What's Your Reaction?
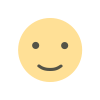
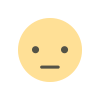
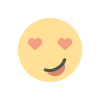
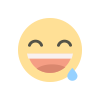
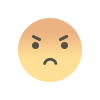
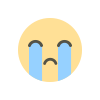
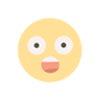