Can language models read the genome? This one decoded mRNA to make better vaccines.
The same class of artificial intelligence that made headlines coding software and passing the bar exam has learned to read a different kind of text — the genetic code. Credit: Photo by Sameer A. Khan/Fotobuddy The same class of artificial intelligence that made headlines coding software and passing the bar exam has learned to read […]
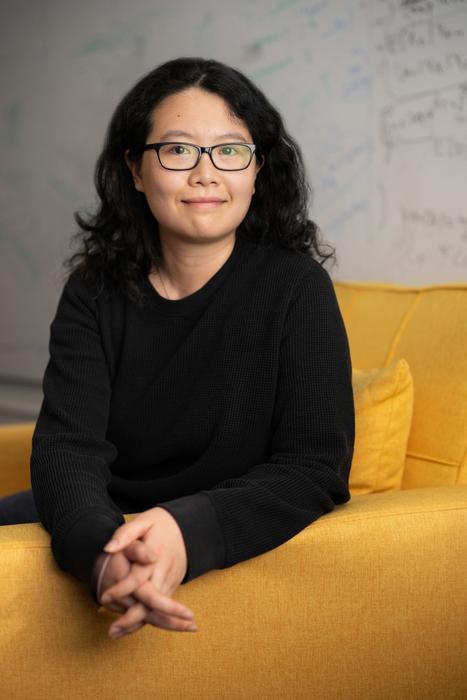
The same class of artificial intelligence that made headlines coding software and passing the bar exam has learned to read a different kind of text — the genetic code.
Credit: Photo by Sameer A. Khan/Fotobuddy
The same class of artificial intelligence that made headlines coding software and passing the bar exam has learned to read a different kind of text — the genetic code.
That code contains instructions for all of life’s functions and follows rules not unlike those that govern human languages. Each sequence in a genome adheres to an intricate grammar and syntax, the structures that give rise to meaning. Just as changing a few words can radically alter the impact of a sentence, small variations in a biological sequence can make a huge difference in the forms that sequence encodes.
Now Princeton University researchers led by machine learning expert Mengdi Wang are using language models to home in on partial genome sequences and optimize those sequences to study biology and improve medicine. And they are already underway.
In a paper published April 5 in the journal Nature Machine Intelligence, the authors detail a language model that used its powers of semantic representation to design a more effective mRNA vaccine such as those used to protect against COVID-19.
Found in Translation
Scientists have a simple way to summarize the flow of genetic information. They call it the central dogma of biology. Information moves from DNA to RNA to proteins. Proteins create the structures and functions of living cells.
Messenger RNA, or mRNA, converts the information into proteins in that final step, called translation. But mRNA is interesting. Only part of it holds the code for the protein. The rest is not translated but controls vital aspects of the translation process.
Governing the efficiency of protein production is a key mechanism by which mRNA vaccines work. The researchers focused their language model there, on the untranslated region, to see how they could optimize efficiency and improve vaccines.
After training the model on a small variety of species, the researchers generated hundreds of new optimized sequences and validated those results through lab experiments. The best sequences outperformed several leading benchmarks for vaccine development, including a 33% increase in the overall efficiency of protein production.
Increasing protein production efficiency by even a small amount provides a major boost for emerging therapeutics, according to the researchers. Beyond COVID-19, mRNA vaccines promise to protect against many infectious diseases and cancers.
Wang, a professor of electrical and computer engineering and the principal investigator in this study, said the model’s success also pointed to a more fundamental possibility. Trained on mRNA from a handful of species, it was able to decode nucleotide sequences and reveal something new about gene regulation. Scientists believe gene regulation, one of life’s most basic functions, holds the key to unlocking the origins of disease and disorder. Language models like this one could provide a new way to probe.
Wang’s collaborators include researchers from the biotech firm RVAC Medicines as well as the Stanford University School of Medicine.
The Language of Disease
The new model differs in degree, not kind, from the large language models that power today’s AI chat bots. Instead of being trained on billions of pages of text from the internet, their model was trained on a few hundred thousand sequences. The model also was trained to incorporate additional knowledge about the production of proteins, including structural and energy-related information.
The research team used the trained model to create a library of 211 new sequences. Each was optimized for a desired function, primarily an increase in the efficiency of translation. Those proteins, like the spike protein targeted by COVID-19 vaccines, drive the immune response to infectious disease.
Previous studies have created language models to decode various biological sequences, including proteins and DNA, but this was the first language model to focus on the untranslated region of mRNA. In addition to a boost in overall efficiency, it was also able to predict how well a sequence would perform at a variety of related tasks.
Wang said the real challenge in creating this language model was in understanding the full context of the available data. Training a model requires not only the raw data with all its features but also the downstream consequences of those features. If a program is designed to filter spam from email, each email it trains on would be labeled “spam” or “not spam.” Along the way, the model develops semantic representations that allow it to determine what sequences of words indicate a “spam” label. Therein lies the meaning.
Wang said looking at one narrow dataset and developing a model around it was not enough to be useful for life scientists. She needed to do something new. Because this model was working at the leading edge of biological understanding, the data she found was all over the place.
“Part of my dataset comes from a study where there are measures for efficiency,” Wang said. “Another part of my dataset comes from another study [that] measured expression levels. We also collected unannotated data from multiple resources.” Organizing those parts into one coherent and robust whole — a multifaceted dataset that she could use to train a sophisticated language model — was a massive challenge.
“Training a model is not only about putting together all those sequences, but also putting together sequences with the labels that have been collected so far. This had never been done before.”
The paper, “A 5′ UTR Language Model for Decoding Untranslated Regions of mRNA and Function Predictions,” was published in Nature Machine Learning. Additional authors include Dan Yu, Yupeng Li, Yue Shen and Jason Zhang, from RVAC Medicines; Le Cong from Stanford; and Yanyi Chu and Kaixuan Huang from Princeton.
Journal
Nature Machine Intelligence
DOI
10.1038/s42256-024-00823-9
Method of Research
Experimental study
Subject of Research
Cells
Article Title
A 5′ UTR Language MoA 5′ UTR Language Model for Decoding Untranslated Regions of mRNA and Function Predictionsdel for Decoding Untranslated Regions of mRNA and Function Predictions
Article Publication Date
5-Apr-2024
What's Your Reaction?
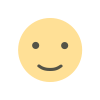
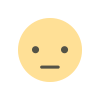
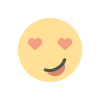
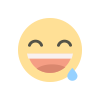
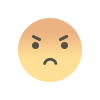
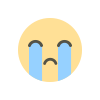
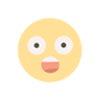