Can a computer tell patients how their multiple sclerosis will progress?
Machine learning models can reliably inform clinicians about the disability progression of multiple sclerosis, according to a new study published this week in the open-access journal PLOS Digital Health by Edward De Brouwer of KU Leuven, Belgium, and colleagues. Credit: De Brouwer et al., 2024, PLOS Digital Health, CC-BY 4.0 (https://creativecommons.org/licenses/by/4.0/) Machine learning models can […]

Machine learning models can reliably inform clinicians about the disability progression of multiple sclerosis, according to a new study published this week in the open-access journal PLOS Digital Health by Edward De Brouwer of KU Leuven, Belgium, and colleagues.
Credit: De Brouwer et al., 2024, PLOS Digital Health, CC-BY 4.0 (https://creativecommons.org/licenses/by/4.0/)
Machine learning models can reliably inform clinicians about the disability progression of multiple sclerosis, according to a new study published this week in the open-access journal PLOS Digital Health by Edward De Brouwer of KU Leuven, Belgium, and colleagues.
Multiple sclerosis (MS) is a chronic progressive autoimmune disease that leads to severe disability over time through a complex pattern of progression, recovery, and relapse. Its global prevalence has increased by more than 30% over the last decade. Yet there are few tools that can predict the progression of MS to help clinicians and patients make life planning and treatment decision-making.
In the new study, De Brouwer and colleagues used data on 15,240 adults with at least three years of MS history who were being treated at 146 MS centers in 40 countries. Data on two years of each patient’s disease progression was used to train state-of-the-art machine learning models to predict the probability of disease progression over the subsequent months and years. The models were trained and validated using strict clinical guidelines, promoting applicability of the models in clinical practice. While individual models varied in performance across different patient subgroups, the models had an average area under the ROC curve (ROC-AUC) of 0.71 ± 0.01. The study found that the history of disability progression was more predictive for future disability progression than treatment or relapse history.
The authors conclude that the models developed in the study have the potential to greatly enhance planning for individuals with MS and could be evaluated in a clinical impact study.
De Brouwer adds: “Using the clinical history of more than 15,000 people with multiple sclerosis, we trained a machine learning model capable of reliably predicting the probability of disability progression in the next two years. The model only uses routinely collected clinical variables, which makes it widely applicable. Our rigorous benchmarking and external validation support the vast potential of machine learning models for helping patients planning their lives and clinicians optimizing treatment strategies.”
############
In your coverage, please use this URL to provide access to the freely available article in PLOS Digital Health: https://journals.plos.org/digitalhealth/article?id=10.1371/journal.pdig.0000533
Citation: De Brouwer E, Becker T, Werthen-Brabants L, Dewulf P, Iliadis D, Dekeyser C, et al. (2024) Machine-learning-based prediction of disability progression in multiple sclerosis: An observational, international, multi-center study. PLOS Digit Health 3(7): e0000533. https://doi.org/10.1371/journal.pdig.0000533
Author Countries: Argentina, Australia, Belgium, Canada, Czech Republic, Greece, Hungary, Italy, Ireland, Kuwait, Lebanon, Malta, The Netherlands, Oman, Spain, Saudi Arabia, Switzerland, Turkey, Romania, United Kingdom, United States
Funding: This study was funded by the Research Foundation Flanders (FWO) and the Flemish government through the Onderzoeksprogramma Artificiele Intelligentie (AI) Vlaanderen program (https://www.flandersairesearch.be/en). This funding was awarded to YM, LB, TD, DD, WW, and BDB and funded EBD, TB, LWB, PD, DI, MS, YM, LB, TD, DD, WW, and BDB. EDB was also concomitantly funded by a FWO-SB fellowship (1S98821N – https://fwo.be). The funders had no role in study design, data collection and analysis, decision to publish, or preparation of the manuscript.
Journal
PLOS Digital Health
DOI
10.1371/journal.pdig.0000533
Method of Research
Computational simulation/modeling
Subject of Research
People
Article Title
Machine-learning-based prediction of disability progression in multiple sclerosis: An observational, international, multi-center study
Article Publication Date
25-Jul-2024
COI Statement
Competing Interests: The authors declare no competing non-financial interests but the following competing financial interests: – Dana Horakova received speaker honoraria and consulting fees from Biogen, Merck, Teva, Roche, Sanofi Genzyme, and Novartis, as well as support for research activities from Biogen and Czech Minsitry of Education [project Progres Q27/LF1]. – Francesco Patti received speaker honoraria and advisory board fees from Almirall, Bayer, Biogen, Celgene, Merck, Novartis, Roche, Sanofi-Genzyme and TEVA. He received research funding from Biogen, Merck, FISM (Fondazione Italiana Sclerosi Multipla), Reload Onlus Association and University of Catania. – Guillermo Izquierdo received speaking honoraria from Biogen, Novartis, Sanofi, Merck, Roche, Almirall and Teva. – Sara Eichau received speaker honoraria and consultant fees from Biogen Idec, Novartis, Merck, Bayer, Sanofi Genzyme, Roche and Teva. – Marc Girard received consulting fees from Teva Canada Innovation, Biogen, Novartis and Genzyme Sanofi; lecture payments from Teva Canada Innovation, Novartis and EMD. He has also received a research grant from Canadian Institutes of Health Research. – Alessandra Lugaresi has served as a Biogen, Bristol Myers Squibb, Merck Serono, Novartis, Roche, Sanofi/ Genzyme and Teva Advisory Board Member. She received congress and travel/accommodation expense compensations or speaker honoraria from Biogen, Merck, Mylan, Novartis, Roche, Sanofi/Genzyme, Teva and Fondazione Italiana Sclerosi Multipla (FISM). Her institutions received research grants from Novartis and Sanofi Genzyme. – Pierre Grammond has served in advisory boards for Novartis, EMD Serono, Roche, Biogen idec, Sanofi Genzyme, Pendopharm and has received grant support from Genzyme and Roche, has received research grants for his institution from Biogen idec, Sanofi Genzyme, EMD Serono. – Tomas Kalincik served on scientific advisory boards for BMS, Roche, Janssen, Sanofi Genzyme, Novartis, Merck and Biogen, steering committee for Brain Atrophy Initiative by Sanofi Genzyme, received conference travel support and/or speaker honoraria from WebMD Global, Eisai, Novartis, Biogen, Sanofi-Genzyme, Teva, BioCSL and Merck and received research or educational event support from Biogen, Novartis, Genzyme, Roche, Celgene and Merck. – Raed Alroughani received honoraria as a speaker and for serving on scientific advisory boards from Bayer, Biogen, GSK, Merck, Novartis, Roche and Sanofi-Genzyme. – Francois Grand’Maison received honoraria or research funding from Biogen, Genzyme, Novartis, Teva Neurosciences, Mitsubishi and ONO Pharmaceuticals. – Murat Terzi received travel grants from Novartis, Bayer-Schering, Merck and Teva; has participated in clinical trials by Sanofi Aventis, Roche and Novartis. – Jeannette Lechner-Scott travel compensation from Novartis, Biogen, Roche and Merck. Her institution receives the honoraria for talks and advisory board commitment as well as research grants from Biogen, Merck, Roche, TEVA and Novartis. – Samia J. Khoury received compensation for participation in the Novartis Maestro program. – Vincent van Pesch has received travel grants from Merck, Biogen, Sanofi, Bristol Myers Squibb, Almirall and Roche; his institution receives honoraria for consultancy and lectures and research grants from Roche, Biogen, Sanofi, Merck, Bristol Myers Squibb, Janssen, Almirall and Novartis Pharma. – Radek Ampapa received conference travel support from Novartis, Teva, Biogen, Bayer and Merck and has participated in a clinical trials by Biogen, Novartis, Teva and Actelion. – Daniele Spitaleri received honoraria as a consultant on scientific advisory boards by Bayer-Schering, Novartis and Sanofi-Aventis and compensation for travel from Novartis, Biogen, Sanofi Aventis, Teva and Merck. – Claudio Solaro served on scientific advisory boards for Merck, Genzyme, Almirall, and Biogen; received honoraria and travel grants from Sanofi Aventis, Novartis, Biogen, Merck, Genzyme and Teva. – Davide Maimone served on scientific advisory boards for Bayer, Biogen, Merck, Sanofi-Genzyme, Novartis, Roche, and Almirall; received honoraria and travel grants from Sanofi Genzyme, Novartis, Biogen, Merck, and Roche. – Gerardo Iuliano (retired – no PI successor but has approved ongoing use of data) had travel/accommodations/meeting expenses funded by Bayer Schering, Biogen, Merck, Novartis, Sanofi Aventis, and Teva. – Bart Van Wijmeersch received research and travel grants, honoraria for MS-Expert advisor and Speaker fees from Bayer-Schering, Biogen, Sanofi Genzyme, Merck, Novartis, Roche and Teva. – Tamara Castillo Triviño received speaking/consulting fees and/or travel funding from Bayer, Biogen, Merck, Novartis, Roche, Sanofi-Genzyme and Teva. – Jose Luis Sanchez-Menoyo accepted travel compensation from Novartis, Merck and Biogen, speaking honoraria from Biogen, Novartis, Sanofi, Merck, Almirall, Bayer and Teva and has participated in clinical trials by Biogen, Merck and Roche – Guy Laureys received travel and/or consultancy compensation from Sanofi-Genzyme, Roche, Teva, Merck, Novartis, Celgene, Biogen. – Anneke van der Walt served on advisory boards and receives unrestricted research grants from Novartis, Biogen, Merck and Roche She has received speaker’s honoraria and travel support from Novartis, Roche, and Merck. She receives grant support from the National Health and Medical Research Council of Australia and MS Research Australia. – Jiwon Oh has received research funding from the MS Society of Canada, National MS Society, Brain Canada, Biogen, Roche, EMD Serono (an affiliate of Merck KGaA); and personal compensation for consulting or speaking from Alexion, Biogen, Celgene (BMS), EMD Serono (an affiliate of Merck KGaA), Novartis, Roche, and Sanofi-Genzyme. – Ayse Altintas received speaker honoraria from Merck, Alexion,; received travel and registration grants from Merck, Biogen – Gen Pharma, Roche, Sanofi-Genzyme. – Yara Fragoso received honoraria as a consultant on scientific advisory boards by Novartis, Teva, Roche and Sanofi-Aventis and compensation for travel from Novartis, Biogen, Sanofi Aventis, Teva, Roche and Merck. – Tunde Csepany received speaker honoraria/ conference travel support from Bayer Schering, Biogen, Merck, Novartis, Roche, Sanofi-Aventis and Teva. – Suzanne Hodgkinson received honoraria and consulting fees from Novartis, Bayer Schering and Sanofi, and travel grants from Novartis, Biogen Idec and Bayer Schering. – Norma Deri received funding from Bayer, Merck, Biogen, Genzyme and Novartis. – Bruce Taylor received funding for travel and speaker honoraria from Bayer Schering Pharma, CSL Australia, Biogen and Novartis, and has served on advisory boards for Biogen, Novartis, Roche and CSL Australia. – Fraser Moore participated in clinical trials sponsored by EMD Serono and Novartis. – Orla Gray received honoraria as consultant on scientific advisory boards for Genzyme, Biogen, Merck, Roche and Novartis; has received travel grants from Biogen, Merck, Roche and Novartis; has participated in clinical trials by Biogen and Merck. – Csilla Rozsa received speaker honoraria from Bayer Schering, Novartis and Biogen, congress and travel expense compensations from Biogen, Teva, Merck and Bayer Schering. – Allan Kermode received speaker honoraria and scientific advisory board fees from Bayer, BioCSL, Biogen, Genzyme, Innate Immunotherapeutics, Merck, Novartis, Sanofi, Sanofi-Aventis, and Teva. – Magdolna Simo received speaker honoraria from Novartis, Biogen, Bayer Schering; congress/travel compensation from Teva, Biogen, Merck, Bayer Schering. – Todd Hardy has received speaking fees or received honoraria for serving on advisory boards for Biogen, Merck, Teva, Novartis, Roche, Bristol-Myers Squibb and Sanofi-Genzyme, is Co-Editor of Advances in Clinical Neurosciences and Rehabilitation, and serves on the editorial board of Journal of Neuroimmunology and Frontiers in Neurology. – Nikolaos Grigoriadis received honoraria, consultancy/lecture fees, travel support and research grants from Biogen Idec, Biologix, Novartis, TEVA, Bayer, Merck Serono, Genesis Pharma, Sanofi – Genzyme, ROCHE, Cellgene, ELPEN and research grants from Hellenic Ministry of Development.
What's Your Reaction?
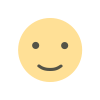
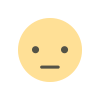
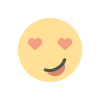
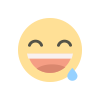
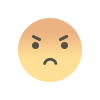
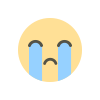
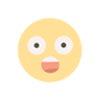