Breakthrough in Precision Agriculture: New Spectral Model Enhances Soybean Detection
In a landmark advancement for global agricultural monitoring, researchers have developed a novel technique that dramatically enhances the precision of soybean mapping worldwide. The innovative method, known as Spectral Gaussian Mixture Modeling (SGMM), leverages the intricate physiological and spectral characteristics of soybean plants to deliver unprecedented accuracy across diverse geographic regions. This breakthrough promises to […]

In a landmark advancement for global agricultural monitoring, researchers have developed a novel technique that dramatically enhances the precision of soybean mapping worldwide. The innovative method, known as Spectral Gaussian Mixture Modeling (SGMM), leverages the intricate physiological and spectral characteristics of soybean plants to deliver unprecedented accuracy across diverse geographic regions. This breakthrough promises to transform how scientists, farmers, and policymakers track and manage one of the world’s most vital crops.
Soybean, a cornerstone crop feeding billions and underpinning industries from food production to biofuels, has long posed significant challenges for accurate remote sensing classification. Traditional mapping techniques frequently falter because of regional climate disparities, phenological variations, and the spectral similarity of soybeans to other crops. Previous machine learning approaches, though powerful, often depend heavily on large, labeled datasets and struggle to generalize well across varied environmental conditions. SGMM surmounts these obstacles by introducing a probabilistic framework rooted in spectral data analysis that effectively captures the temporal and spatial nuances of soybean growth.
At the heart of this paradigm shift is the SGMM’s ability to dynamically adjust classification parameters according to regional and temporal variations. Unlike rigid threshold-based models, SGMM embraces uncertainty through Gaussian mixtures that represent the complex spectral signatures of vegetation within a given pixel. Central to its mechanism is the calculation of the Optimal Time Window (OTW), a period during the soybean growth cycle in which spectral features—such as the Shortwave Infrared 1 (SWIR1), Enhanced Vegetation Index (EVI), and Green Chlorophyll Vegetation Index (GCVI)—are most discriminative. Focusing on this window significantly mitigates classification errors stemming from phenological shifts.
One of the key innovations is the incorporation of the Bhattacharyya Coefficient (BC) as a weighting factor within the model. The BC quantifies the statistical similarity between soybean and non-soybean spectral distributions, effectively serving as a penalty to reduce false positives and negatives. This integration fine-tunes the mixture components, ensuring that subtle spectral overlaps do not mislead the classifier. By optimizing spectral separability in this fashion, SGMM achieves a robust delineation of soybean fields even in regions where other crops or natural vegetation exhibit similar reflectance properties.
The research team rigorously validated the SGMM approach across four major soybean-producing countries—China, the United States, Argentina, and Brazil—each with markedly different climates, soil types, and agricultural practices. Across these varied landscapes, SGMM consistently delivered classification accuracies between 87.5% and 90.7%, a quantum leap over prevailing techniques. This impressive performance was further substantiated by showing strong correlations between provincial-level SGMM-generated maps and official agricultural census statistics, underscoring the model’s practical reliability and scalability.
Traditional remote sensing methods often encounter difficulties due to cloud cover, soil background variability, and atmospheric disturbance, which degrade image quality and spectral clarity. SGMM addresses these concerns by incorporating spectral uncertainty directly into its Gaussian mixture components and selecting the OTW with the highest data quality. Moreover, the model’s architecture allows integration with multi-temporal satellite datasets, enhancing resilience against gaps caused by transient weather conditions. Collectively, these strengths position SGMM as a forward-looking tool for precision agriculture amidst dynamic environmental challenges.
Another remarkable aspect of SGMM is its computational efficiency and reduced reliance on massive labeled datasets. Common deep learning frameworks necessitate extensive ground-truthing for training, a resource-intensive and time-consuming burden. In contrast, SGMM’s probabilistic modeling framework requires fewer labeled samples, relying instead on the underlying statistical properties of spectral data to generalize classification across regions. This efficiency opens the door to rapid deployment in emerging agricultural frontiers where data scarcity and diverse cropping systems have traditionally impeded remote sensing applications.
Lead scientist Dr. Shuangxi Miao highlighted the transformative potential of the SGMM framework: “By combining spectral feature optimization with probabilistic modeling, SGMM addresses the long-standing challenges of regional inconsistency and phenological variability in soybean mapping. This approach not only boosts classification accuracy but also delivers the scalability needed for global monitoring, essential for tackling food security in our rapidly changing world.” His statement underscores the model’s ability to provide stakeholders with timely, actionable information crucial for decision-making and resource management.
Beyond soybeans, the flexibility of the SGMM architecture suggests broad applicability to other staple crops such as maize and wheat, whose spectral characteristics also fluctuate with environmental conditions and development stages. Integrating real-time satellite data streams, SGMM could be tailored to improve the accuracy of crop yield predictions, detect stress events earlier, and optimize supply chains across global agricultural systems. This adaptability heralds a new era in remote sensing-based agronomy and sustainable intensification.
Looking forward, the research team is exploring the fusion of SGMM with advanced artificial intelligence techniques to further enhance performance, particularly in complex agricultural scenarios such as intercropping systems and regions with persistent cloud cover. By refining spectral feature extraction algorithms and incorporating ancillary data sources, future iterations of the model aim to overcome current limitations and unlock higher-resolution, real-time crop monitoring capabilities on a planetary scale.
The publication of this breakthrough comes at a critical time when global food security faces mounting pressures from climate change, population growth, and shifting dietary demands. SGMM’s precision and generalizability offer a powerful tool for governments, agribusinesses, and researchers striving to optimize crop management practices and ensure sustainable food production. Its potential to integrate seamlessly with existing agricultural intelligence systems makes it an indispensable asset in the global endeavor to feed billions efficiently.
In summary, Spectral Gaussian Mixture Modeling represents a seminal advance in remote sensing methodology, imbuing soybean mapping with unmatched accuracy, adaptability, and scalability. By combining rigorous spectral analysis with probabilistic machine learning, this approach transcends the limitations of traditional crop classification models. Its success across multiple continents signals a promising future where high-resolution, real-time agricultural monitoring becomes the standard, empowering stakeholders to meet the challenges of the 21st century with data-driven precision.
Subject of Research:
Agriculture, Remote Sensing, Crop Science, Soybean Mapping
Article Title:
Improved Soybean Mapping with Spectral Gaussian Mixture Modeling
News Publication Date:
17-Apr-2025
References:
DOI: 10.34133/remotesensing.0473
Image Credits:
Journal of Remote Sensing
Keywords:
Soybeans, Spectral Gaussian Mixture Model, Remote Sensing, Crop Mapping, Precision Agriculture, Machine Learning, Vegetation Indices, Bhattacharyya Coefficient, Optimal Time Window
Tags: agricultural monitoring advancementsagricultural policy implicationscrop mapping accuracyinnovative farming technologiesmachine learning in agricultureprecision agricultureregional climate impact on cropsremote sensing classification challengessoybean mapping technologyspectral data analysis techniquesSpectral Gaussian Mixture Modelingtemporal and spatial variations in agriculture
What's Your Reaction?
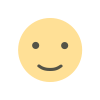
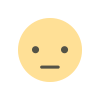
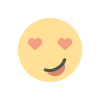
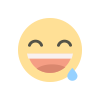
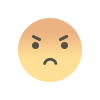
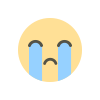
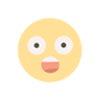