Breakthrough in Atrial Fibrillation Treatment: Synthetic Scarred Hearts Show Promise
In an innovative leap towards personalized medicine, researchers at Queen Mary University of London have unveiled a groundbreaking artificial intelligence (AI) tool designed specifically for the creation of synthetic, yet medically accurate models of fibrotic heart tissue. This development is particularly aimed at enhancing treatment planning for patients suffering from atrial fibrillation (AF), a prevalent […]

In an innovative leap towards personalized medicine, researchers at Queen Mary University of London have unveiled a groundbreaking artificial intelligence (AI) tool designed specifically for the creation of synthetic, yet medically accurate models of fibrotic heart tissue. This development is particularly aimed at enhancing treatment planning for patients suffering from atrial fibrillation (AF), a prevalent heart rhythm disorder affecting millions. The findings, published in the esteemed journal Frontiers in Cardiovascular Medicine, suggest a transformative shift in how physicians may approach care for AF patients in the near future.
Fibrosis, marked by the development of scar tissue within the heart, can emerge as a consequence of numerous factors, including aging, long-term stress, and the AF condition itself. This fibrous tissue, which is rigid and disrupts the heart’s intricate electrical system, plays a crucial role in the irregular heartbeat frequently observed in AF. The distribution and pattern of this scarring are pivotal in determining the success of various treatment options and are monitored via a specialized imaging technique known as Late Gadolinium Enhancement Magnetic Resonance Imaging, or LGE-MRI.
The conventional treatment for atrial fibrillation often involves a procedure called ablation, where cardiologists strategically create small, controlled scars in order to block erratic electrical signals contributing to the arrhythmia. Yet, despite advancements in surgical technology, success rates remain inconsistent, with ablation procedures failing in nearly half of the cases. The quest for reliable predictors of treatment outcomes remains a significant challenge for healthcare professionals worldwide.
One of the core difficulties in utilizing AI to predict patient outcomes in AF treatment is the scarcity of high-quality imaging data. “While LGE-MRI is invaluable for assessing heart fibrosis, acquiring a substantial enough dataset of scans for effective AI training remains a daunting task,” states Dr. Alexander Zolotarev, the first author of the study and a key figure in the groundbreaking research. In a remarkable feat, the team managed to train their AI model using a mere 100 authentic LGE-MRI scans from AF patients. The result was an impressive generation of 100 additional synthetic fibrosis patterns, each accurately mimicking genuine heart scarring.
These virtual models of fibrotic tissue were then employed to simulate various ablation strategies across diverse patient anatomies. This innovative approach allowed researchers to assess how different methods might yield varying results based on the unique characteristics of each patient’s heart. Remarkably, the predictive reliability of the AI-created patterns was found to be nearly on par with those derived from actual patient data, presenting an exciting benchmark for the future of cardiac treatment planning.
A significant ethical consideration in this research is the maintenance of patient privacy. By utilizing synthetic fibrosis patterns, the researchers can explore a substantially broader range of cardiac scenarios than traditional methods allow, effectively sidestepping the legal and moral complexities associated with using real patient data. This aligns with a growing emphasis in healthcare on the importance of patient confidentiality while still fostering advances in medical technology.
It is important to note that the role of AI in this capacity is not to replace the nuanced judgment of clinical professionals. “Our aim is not about supplanting doctors,” Dr. Zolotarev highlights. “This technology acts as a sophisticated simulator for clinicians, providing them with a platform to experiment with an array of treatment approaches on a digital representation of each patient’s unique heart structure before proceeding with the actual procedure.” This paradigm shift could lead to significantly enhanced predictions of treatment outcomes, ultimately guiding more effective and individualized therapeutic strategies for AF patients.
Additionally, this pioneering study comes as part of a broader initiative led by Dr. Caroline Roney under the UKRI Future Leaders Fellowship program, which targets the creation of personalized ‘digital twin’ heart models for patients afflicted with atrial fibrillation. Dr. Roney expressed enthusiasm about the research, stating that it addresses the pressing issue of limited clinical data availability, a significant hurdle in developing effective cardiac digital twin models.
With approximately 1.4 million individuals in the United Kingdom affected by atrial fibrillation, and with ablation procedures failing in a staggering proportion of cases, the implications of this AI tool are profound. It holds the potential to not only improve predictive accuracy for AF treatments but also significantly diminish the need for repeat procedures, which are not only costly but also carry health risks for patients.
The essence of this research underscores the pivotal role of artificial intelligence as a crucial tool to advance clinical practices in medicine. By providing clinicians with advanced modeling capabilities, this initiative addresses two critical challenges in healthcare: the limited availability of quality patient data and the ethical imperative to safeguard sensitive medical information. As AI technology continues to evolve and integrate into clinical settings, the possibilities for improved patient outcomes are exciting.
This develops a fertile ground for initiating broader in silico trials that facilitate the generation of larger datasets for training AI models, further empowering researchers and practitioners. The future of personalized care for atrial fibrillation may very well lie in these innovative synthetic models, shaping a new standard in patient-specific treatment and paving the path for a new era of heart health management.
The integration of synthetic modeling techniques, coupled with traditional medical assessments, offers a transformative approach to treating cardiac arrhythmias with an emphasis on effectiveness and safety. As the field moves forward, this research exemplifies a significant stride towards achieving a pivotal intersection where cutting-edge technology meets compassionate patient care, promising a brighter future for many.
With the continuing exploration of synthetic fibrosis distributions and their applications in predicting atrial fibrillation ablation outcomes, there lies an exciting horizon for both researchers and patients alike. Collectively, the advancements spearheaded by the team at Queen Mary University of London signify an important milestone in cardiovascular medicine, reinforcing the necessity and potential of marrying artificial intelligence with clinical practice to revolutionize patient treatment paradigms.
The interdisciplinary collaboration between researchers, clinicians, and technologists within this study highlights the importance of collective efforts in addressing complex healthcare challenges. By harnessing the power of AI and embracing new technological innovations, we may unlock invaluable insights into heart conditions, leading to more effective management options for patients grappling with atrial fibrillation and other cardiovascular issues in the future.
Subject of Research: Synthetic fibrosis distributions for predicting atrial fibrillation ablation outcomes.
Article Title: Synthetic fibrosis distributions for data augmentation in predicting atrial fibrillation ablation outcomes: an in silico study.
News Publication Date: 11-Apr-2025.
Web References: Frontiers in Cardiovascular Medicine
References: DOI: 10.3389/fcvm.2025.1512356
Image Credits: None.
Keywords
Atrial fibrillation, synthetic fibrosis, AI in medicine, digital twin models, cardiac arrhythmias, medical imaging, ablation procedures, patient privacy, computational modeling, personalized medicine, heart health management, in silico trials.
Tags: ablation procedure for AFAI in personalized medicineAtrial fibrillation treatment advancementscardiac fibrosis and its effectsfibrotic heart tissue researchinnovative AI tools in cardiologyirregular heartbeat managementLate Gadolinium Enhancement MRIQueen Mary University researchscar tissue and electrical signals in the heartsynthetic heart tissue modelstransformative approaches in heart disease
What's Your Reaction?
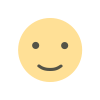
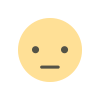
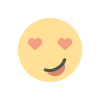
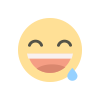
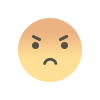
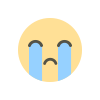
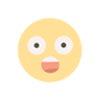