Brain-inspired chaotic spiking backpropagation
This study is led by Mr. Zijian Wang, Dr. Peng Tao and Prof. Luonan Chen at Hangzhou Institute for Advanced Study, University of Chinese Academy of Sciences. Since Freeman et al. discovered in the 1980s that learning in the rabbit brain utilizes chaos, this nonlinear and initially value-sensitive dynamical behavior has been increasingly recognized as […]
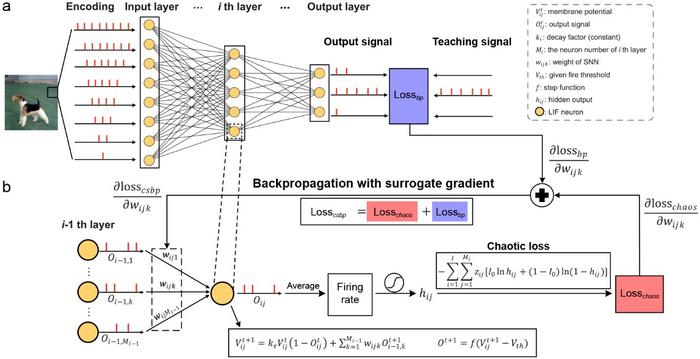
This study is led by Mr. Zijian Wang, Dr. Peng Tao and Prof. Luonan Chen at Hangzhou Institute for Advanced Study, University of Chinese Academy of Sciences. Since Freeman et al. discovered in the 1980s that learning in the rabbit brain utilizes chaos, this nonlinear and initially value-sensitive dynamical behavior has been increasingly recognized as integral to brain learning. However, modern learning algorithms for artificial neural networks, particularly spiking neural networks (SNNs) that closely resemble the brain, have not effectively capitalized on this feature.
Credit: ©Science China Press
This study is led by Mr. Zijian Wang, Dr. Peng Tao and Prof. Luonan Chen at Hangzhou Institute for Advanced Study, University of Chinese Academy of Sciences. Since Freeman et al. discovered in the 1980s that learning in the rabbit brain utilizes chaos, this nonlinear and initially value-sensitive dynamical behavior has been increasingly recognized as integral to brain learning. However, modern learning algorithms for artificial neural networks, particularly spiking neural networks (SNNs) that closely resemble the brain, have not effectively capitalized on this feature.
Zijian Wang and Peng Tao, together with lab director Luonan Chen, endeavored to introduce intrinsic chaotic dynamics in the brain into the learning algorithms of existing SNNs. They found that this could be accomplished by merely integrating a loss function analogous to cross-entropy (see image below). Furthermore, they observed that an SNN equipped with chaotic dynamics not only enhances learning/optimization performance, but also improves the generalization performance on both neuromorphic datasets (e.g., DVS-CIFAR10 and DVS-Gesture) and large-scale static datasets (e.g., CIFAR100 and ImageNet), with the help of the ergodic and pseudo-random properties of chaos.
The team also experimented with the introduction of extrinsic chaos, such as by using Logistic maps. However, this did not enhance the learning performance of SNNs. “This is an exciting result, and it implies that the intrinsic chaos that the brain has evolved over billions of years plays important roles in its learning efficiency.” Luonan Chen says.
Although SNNs have stronger spatio-temporal signal characterization and higher energy utilization efficiency, their performance often lags behind that of traditional neural networks of equivalent size due to the lack of efficient learning algorithms. This new algorithm effectively bridges this gap. Moreover, since only one additional loss function needs to be introduced, it can be employed as a generalized plug-in unit with existing SNN learning methodologies.
See the article:
Brain-inspired Chaotic Spiking Backpropagation
https://doi.org/10.1093/nsr/nwae037
Journal
National Science Review
DOI
10.1093/nsr/nwae037
What's Your Reaction?
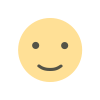
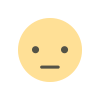
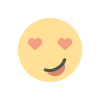
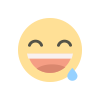
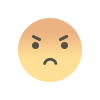
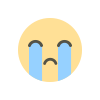
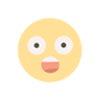