Ant insights lead to robot navigation breakthrough
Have you ever wondered how insects are able to go so far beyond their home and still find their way? The answer to this question is not only relevant to biology but also to making the AI for tiny, autonomous robots. TU Delft drone-researchers felt inspired by biological findings on how ants visually recognize their […]

Have you ever wondered how insects are able to go so far beyond their home and still find their way? The answer to this question is not only relevant to biology but also to making the AI for tiny, autonomous robots. TU Delft drone-researchers felt inspired by biological findings on how ants visually recognize their environment and combine it with counting their steps in order to get safely back home. They have used these insights to create an insect-inspired autonomous navigation strategy for tiny, lightweight robots. The strategy allows such robots to come back home after long trajectories, while requiring extremely little computation and memory (0.65 kiloByte per 100 m). In the future, tiny autonomous robots could find a wide range of uses, from monitoring stock in warehouses to finding gas leaks in industrial sites. The researchers have published their findings in Science Robotics, on July 17, 2024.
Credit: Guido de Croon / TU Delft|MAV Lab
Have you ever wondered how insects are able to go so far beyond their home and still find their way? The answer to this question is not only relevant to biology but also to making the AI for tiny, autonomous robots. TU Delft drone-researchers felt inspired by biological findings on how ants visually recognize their environment and combine it with counting their steps in order to get safely back home. They have used these insights to create an insect-inspired autonomous navigation strategy for tiny, lightweight robots. The strategy allows such robots to come back home after long trajectories, while requiring extremely little computation and memory (0.65 kiloByte per 100 m). In the future, tiny autonomous robots could find a wide range of uses, from monitoring stock in warehouses to finding gas leaks in industrial sites. The researchers have published their findings in Science Robotics, on July 17, 2024.
Sticking up for the little guy
Tiny robots, from tens to a few hundred grams, have the potential for interesting real-world applications. With their light weight, they are extremely safe even if they accidentally bump into someone. Since they are small, they can navigate in narrow areas. And if they can be made cheaply, they can be deployed in larger numbers, so that they can quickly cover a large area, for instance in greenhouses for early pest or disease detection.
However, making such tiny robots operate by themselves is difficult, since compared to larger robots they have extremely limited resources. A major obstacle is that they have to be able to navigate by themselves. For this robots can get help from external infrastructure. They can use location estimates from GPS satellites outdoors or from wireless communication beacons indoors. However, it is often not desirable to rely on such infrastructure. GPS is unavailable indoors and can get highly inaccurate in cluttered environments such as in urban canyons. And installing and maintaining beacons in indoor spaces is quite expensive or simply not possible, for example in search-and-rescue scenarios.
The AI necessary for autonomous navigation with only onboard resources has been made with large robots in mind such as self-driving cars. Some approaches rely on heavy, power-hungry sensors like LiDAR laser rangers, which can simply not be carried or powered by small robots. Other approaches use the sense of vision, which is a very power-efficient sensor that provides rich information on the environment. However, these approaches typically attempt to create highly detailed 3D maps of the environment. This requires large amounts of processing and memory, which can only be provided by computers that are too large and power-hungry for tiny robots.
Counting steps and visual breadcrumbs
This is why some researchers have turned to nature for inspiration. Insects are especially interesting as they operate over distances that could be relevant to many real-world applications, while using very scarce sensing and computing resources. Biologists have an increasing understanding of the underlying strategies used by insects. Specifically, insects combine keeping track of their own motion (termed “odometry”) with visually guided behaviors based on their low-resolution, but almost omnidirectional visual system (termed “view memory”). Whereas odometry is increasingly well understood even up to the neuronal level, the precise mechanisms underlying view memory are still less well understood. One of the earliest theories on how this works proposes a “snapshot” model. In it, an insect such as an ant is proposed to occasionally make snapshots of its environment. Later, when arriving close to the snapshot, the insect can compare its current visual percept to the snapshot, and move to minimize the differences. This allows the insect to navigate, or ‘home’, to the snapshot location, removing any drift that inevitably builds up when only performing odometry.
“Snapshot-based navigation can be compared to how Hansel tried not to get lost in the fairy tale of Hansel and Gretel. When Hans threw stones on the ground, he could get back home. However, when he threw bread crumbs that were eaten by the birds, Hans and Gretel got lost. In our case, the stones are the snapshots.” says Tom van Dijk, first author of the study, “As with a stone, for a snapshot to work, the robot has to be close enough to the snapshot location. If the visual surroundings get too different from that at the snapshot location, the robot may move in the wrong direction and never get back anymore. Hence, one has to use enough snapshots – or in the case of Hansel drop a sufficient number of stones. On the other hand, dropping stones to close to each other would deplete Hans’ stones too quickly. In the case of a robot, using too many snapshots leads to large memory consumption. Previous works in this field typically had the snapshots very close together, so that the robot could first visually home to one snapshot and then to the next.”
“The main insight underlying our strategy is that you can space snapshots much further apart, if the robot travels between snapshots based on odometry.”, says Guido de Croon, Full Professor in bio-inspired drones and co-author of the article, “Homing will work as long as the robot ends up close enough to the snapshot location, i.e., as long as the robot’s odometry drift falls within the snapshot’s catchment area. This also allows the robot to travel much further, as the robot flies much slower when homing to a snapshot than when flying from one snapshot to the next based on odometry.”
The proposed insect-inspired navigation strategy allowed a 56-gram “CrazyFlie” drone, equipped with an omnidirectional camera, to cover distances of up to 100 meters with only 0.65 kiloByte. All visual processing happened on a tiny computer called a “micro-controller”, which can be found in many cheap electronic devices.
Putting robot technology to work
“The proposed insect-inspired navigation strategy is an important step on the way to applying tiny autonomous robots in the real world.”, says Guido de Croon, “The functionality of the proposed strategy is more limited than that provided by state-of-the-art navigation methods. It does not generate a map and only allows the robot to come back to the starting point. Still, for many applications this may be more than enough. For instance, for stock tracking in warehouses or crop monitoring in greenhouses, drones could fly out, gather data and then return to the base station. They could store mission-relevant images on a small SD card for post-processing by a server. But they would not need them for navigation itself.”
Journal
Science Robotics
DOI
10.1126/scirobotics.adk0310
Method of Research
Experimental study
Subject of Research
Not applicable
Article Title
Visual Route-following for Tiny Autonomous Robots
Article Publication Date
17-Jul-2024
What's Your Reaction?
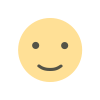
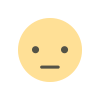
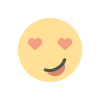
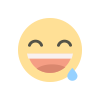
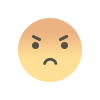
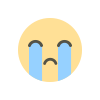
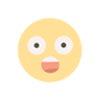