AI Vision Transformer Advances Oral Dysplasia Diagnosis
In a groundbreaking leap towards revolutionizing medical diagnostics, researchers have unveiled an advanced artificial intelligence (AI) model that promises to transform the way oral epithelial dysplasia is detected and graded. Oral epithelial dysplasia (OED) is a precancerous condition marked by abnormal cellular behavior in the oral mucosa, which carries significant implications for oral cancer progression. […]

In a groundbreaking leap towards revolutionizing medical diagnostics, researchers have unveiled an advanced artificial intelligence (AI) model that promises to transform the way oral epithelial dysplasia is detected and graded. Oral epithelial dysplasia (OED) is a precancerous condition marked by abnormal cellular behavior in the oral mucosa, which carries significant implications for oral cancer progression. Accurate grading of this condition through histopathological examination is crucial for timely intervention, yet remains a complex and subjective task, dependent on pathologist expertise. The newly developed system harnesses the power of Vision Transformer (ViT) architecture, a nascent yet highly influential AI model, to analyze intricate tissue images, exhibiting superior performance over traditional convolutional neural networks (CNNs).
Histopathology relies on microscopic examination of stained tissue sections, a process that demands considerable experience and can be prone to inter-observer variability. By automating this analytical workflow, the research team from Tehran University of Medical Sciences aims to augment diagnostic precision while reducing human error and resource burden. The study meticulously collected 218 histopathological slide images from institutional archives complemented by data from publicly accessible repositories. These images were expertly annotated by two independent oral pathologists according to the latest 2022 World Health Organization (WHO) grading system encompassing mild, moderate, and severe dysplasia, in addition to a binary high-risk and low-risk grading classification and a separate category for normal tissue.
The technical heart of this advancement lies within the Vision Transformer algorithm, a paradigm shift from conventional CNN-based deep learning architectures widely used for image classification tasks. Unlike CNNs, which primarily rely on convolution operations to extract local spatial features, Transformers utilize self-attention mechanisms to capture long-range dependencies across the entire image, enabling more holistic and contextual understanding of the complex histological structures present in OED. To benchmark their algorithm’s effectiveness, the researchers compared ViT’s performance against two established CNN models: VGG16 and a custom-built ConvNet.
Data preprocessing formed a critical step, where the raw histopathological slides were segmented into numerous ‘patches’ representing localized tissue regions. This segmentation resulted in a comprehensive dataset comprising 2,545 patches representing low-risk tissues, 2,054 corresponding to high-risk lesions, with further sub-classifications into mild (726 patches), moderate (831), and severe dysplastic tissues (449), along with 937 normal tissue patches. This high-resolution granularity facilitated rigorous model training, testing, and validation, ensuring robustness and generalizability of the developed AI framework.
Quantitative evaluation revealed the remarkable predictive power of the ViT model. In the three-class classification scenario aligning with WHO grading, ViT achieved an accuracy of 94%, significantly outstripping the accuracies of 86% and 88% demonstrated by VGG16 and ConvNet respectively. The model’s superiority was even more pronounced in the four-class scenario integrating the binary classification along with the normal tissue class, where ViT soared to an impressive 97% accuracy, overshadowing VGG16’s 79% and ConvNet’s 88%. These statistics underscore ViT’s advanced capacity to discern subtle morphological variations underlying diverse dysplastic grades.
Beyond raw performance metrics, the study illuminates the transformative impact of integrating Vision Transformers into pathological workflows. ViT’s ability to model global spatial relations allowed for nuanced differentiation of cellular atypia, architectural disruptions, and stromal alterations that are hallmarks of the dysplasia spectrum. This level of interpretability and accuracy surpasses traditional algorithms, empowering AI to not merely supplement but potentially augment pathologist assessments through comprehensive and expedited image analysis.
The implications of this research extend far beyond the immediate domain of oral pathology. With oral cancers ranking among the most incident malignancies globally, early detection of pre-malignant changes represents a vital public health objective. The AI-driven diagnostic approach demonstrated here paves a scalable path towards integrating digital pathology into routine clinical practice—enabling faster, reproducible, and more objective interpretations, especially in settings lacking specialized expertise.
Moreover, this study highlights an exciting frontier where emerging AI models originally conceived for natural language processing and broader computer vision applications are ingeniously repurposed for the biomedical landscape. Vision Transformers, known for their breakthrough results in image recognition benchmarks, have shown remarkable adaptability in handling heterogeneous and complex medical images. Their deployment in OED grading signals a new era of cross-disciplinary synergy, merging computational advances with pathology expertise to revolutionize patient care.
While the findings are undeniably promising, the authors acknowledge challenges ahead before widespread clinical adoption. Validation on larger, multi-institutional datasets, incorporation of diverse staining protocols, and establishing standardized deployment pipelines are essential next steps. Additionally, integrating AI decisions within transparent, interpretable frameworks will be paramount to gain clinician trust and ensure ethical medical practice.
Nevertheless, this research represents a watershed moment in AI-assisted histopathology. By achieving near-human-level accuracy in a notoriously challenging diagnostic category, the Vision Transformer-based approach validates the potential of cutting-edge AI to serve as an independent or complementary diagnostic tool. This not only accelerates the grading process but also democratizes high-quality diagnostics to underserved regions, potentially curtailing the global oral cancer burden.
In summary, the study vividly illustrates how AI innovations can surmount traditional limitations in medical imaging analysis. The Vision Transformer model’s unprecedented accuracy and contextual awareness empower healthcare professionals with enhanced diagnostic capabilities, marking a paradigm shift towards precision oral medicine. Ongoing advancements and collaborative efforts herald a future where AI-driven histopathology integrates seamlessly into clinical pathways, fostering early detection and improving patient prognoses worldwide.
The successful application of Vision Transformers also sets a precedent for exploring other challenging histopathological entities, moving beyond OED towards comprehensive cancer characterization, prognostication, and personalized treatment planning. As this technology matures, synergizing AI with molecular and genomic data could redefine diagnostic algorithms, catalyzing precision oncology.
Ultimately, the convergence of artificial intelligence and pathology heralds a transformative chapter in medical diagnostics. Harnessing Vision Transformers to decode microscopic tissue morphology exemplifies the untapped potential within AI research to elevate healthcare delivery. The present study is a testament to the impactful fusion of computational breakthroughs with clinical acumen, charting a promising course for next-generation diagnostic tools that are faster, smarter, and accessible to all.
Subject of Research: Artificial intelligence application in grading histopathological images of oral epithelial dysplasia using Vision Transformer deep learning algorithms.
Article Title: Artificial intelligence based vision transformer application for grading histopathological images of oral epithelial dysplasia: a step towards AI-driven diagnosis.
Article References: Hadilou, M., Mahdavi, N., Keykha, E. et al. Artificial intelligence based vision transformer application for grading histopathological images of oral epithelial dysplasia: a step towards AI-driven diagnosis. BMC Cancer 25, 780 (2025). https://doi.org/10.1186/s12885-025-14193-x
Image Credits: Scienmag.com
DOI: https://doi.org/10.1186/s12885-025-14193-x
Tags: advanced AI models in healthcareAI in medical diagnosticsAI performance in tissue image analysisautomated diagnostic systems in medicinehistopathological examination automationimproving precision in oral pathologyinter-observer variability in pathologymachine learning for cancer detectionoral cancer precursors diagnosisoral epithelial dysplasia detectionTehran University research in AIVision Transformer for pathology
What's Your Reaction?
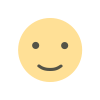
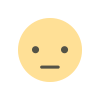
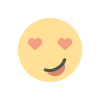
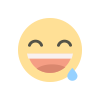
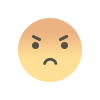
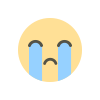
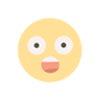