AI-Powered Solutions: Enhancing Suicide Risk Identification for Healthcare Professionals
A groundbreaking study conducted by a team at Vanderbilt University Medical Center offers new hope in the battle against suicide risk in clinical settings through the innovative use of artificial intelligence (AI). The research focuses on how AI-driven clinical alerts can enhance the ability of healthcare professionals to identify patients who may be at risk […]

A groundbreaking study conducted by a team at Vanderbilt University Medical Center offers new hope in the battle against suicide risk in clinical settings through the innovative use of artificial intelligence (AI). The research focuses on how AI-driven clinical alerts can enhance the ability of healthcare professionals to identify patients who may be at risk for suicide, a significant public health challenge that has seen increasing prevalence in recent years. As suicide emerges as a pressing issue, the need for effective screening and intervention methods becomes paramount, making this research particularly timely and relevant.
The principal investigator of this significant endeavor, Dr. Colin Walsh, MD, MA, an associate professor of Biomedical Informatics, Medicine, and Psychiatry at Vanderbilt, heads a dedicated team that embarked on testing their AI system, dubbed the Vanderbilt Suicide Attempt and Ideation Likelihood model (VSAIL). With remarkable foresight, Dr. Walsh and his colleagues aimed to evaluate whether the AI system could effectively prompt healthcare providers in neurology clinics to conduct essential screenings for suicide risk. This approach reflects a proactive strategy to integrate cutting-edge technology into the realm of mental health assessments, ultimately aiming to save lives.
The study, which is set to be published in the peer-reviewed journal JAMA Network Open, meticulously compares two distinct alert mechanisms: the disruptive automatic pop-up alerts versus a more passive display of risk information within the patient’s electronic health record (EHR). This experimental design is not merely a technical exercise; it embodies a crucial step toward improving clinical outcomes by systematically analyzing the ramifications of AI on clinical decision-making processes, particularly in the context of suicide risk assessment.
A noteworthy finding from the research underscores the critical importance of alert design; the study revealed that the interruptive alerts were significantly more effective in prompting doctors to proceed with suicide risk assessments than the passive system. Specifically, the interruptive alerts led to assessments being conducted in response to 42% of trigger notifications, while the passive display of information resulted in only a 4% compliance rate. This stark contrast emphasizes the necessity for timely and attention-catching interventions to drive practitioner response and underscores the potential of AI to reshape clinical workflows.
Dr. Walsh’s observations regarding the relationship between healthcare visits and suicide indicate the complexities underlying this epidemic. He notes that many individuals who tragically die by suicide have engaged with healthcare providers for various reasons unrelated to mental health issues. This observation calls for a strategic pivot in how the medical community approaches patient screening, revealing that universal screening may not be pragmatically achievable in every clinical environment. The development of focused tools like VSAIL seeks to bridge this gap, enabling practitioners to identify high-risk patients more effectively and instigate targeted discussions about mental health.
The statistics surrounding suicide in the United States paint a grim picture: suicide ranks as the 11th leading cause of death, claiming approximately 14.2 lives per 100,000 individuals annually. Alarmingly, research indicates that 77% of patients who die by suicide had interacted with primary care providers within a year preceding their death. This data illustrates the crucial window of opportunity that healthcare settings represent for preventive efforts. The VSAIL model’s innovative data analysis, which utilizes routine EHR information to calculate a patient’s risk of a suicide attempt over the subsequent 30 days, stands as a beacon of hope amidst these concerning statistics.
Through earlier prospective tests of the VSAIL model—where patients were flagged without triggering alerts—the team noted that a disturbing one in 23 individuals identified by the system expressed suicidal thoughts during follow-up assessments. Such evidence underscores the model’s potential utility in clinical practice and solidifies the rationale for implementing AI-derived risk detection strategies. The current study was designed to delve further into this critical issue, leveraging randomized alert systems to gauge their impact on screening adherence in neurology clinics, where certain conditions correlate with heightened risk for suicide.
Importantly, the researchers are advocating for the applicability of AI-driven screening tools across other medical settings, expanding the potential of the VSAIL model beyond neurology. The innovative nature of this research lies not merely in the design of the AI system itself but also in the multifaceted consideration of clinical workflow and patient interaction. With busy care environments often inundated with competing demands, the selective alert strategy employed by VSAIL is crucial. By flagging only 8% of patient visits for screening, the model strikes a balance that enhances the feasibility of integrating mental health evaluations into the routine practice of healthcare providers.
Nonetheless, the journey to implement such innovative approaches is not without challenges. The study did reveal potential drawbacks associated with the use of interruptive alerts, particularly the risk of “alert fatigue,” wherein providers may become desensitized to frequent automated notifications. Addressing this concern will be essential in ensuring that healthcare systems can effectively harness the benefits of AI while maintaining optimal clinician engagement. The researchers stress the importance of balancing alert effectiveness against potential downsides, advocating for well-designed alert mechanisms that sustain clinician responsiveness without overwhelming them.
The implications of this research extend well beyond the immediate findings. As the field of AI in healthcare continues to grow, embracing similar models could play a vital role in reshaping mental health care strategies across various specialties. The success of the VSAIL model at Vanderbilt underscores the transformative potential of AI in filter critical health information for clinicians, thus paving the way for more informed, timely clinical decisions in real-world settings. As suicide prevention remains a vital area of research, the importance of early identification and intervention cannot be overstated, reiterating the key role technology can play in safeguarding patient lives.
In conclusion, this groundbreaking study from Vanderbilt University Medical Center marks a significant advancement in the utilization of AI to combat the public health crisis of suicide. The findings underscore the practicality and necessity for effective, technology-driven solutions that can elevate the standard of care in mental health assessments. As healthcare systems navigate the complexities of patient care, the lessons drawn from VSAIL’s implementation may offer a roadmap for harnessing AI’s full potential in clinical settings, ultimately ushering in a new era of preventive healthcare.
Subject of Research: People
Article Title: Risk Model–Guided Clinical Decision Support for Suicide Screening
News Publication Date: 3-Jan-2025
Web References: DOI
References: N/A
Image Credits: Credit: Vanderbilt University Medical Center
Keywords: Suicide, Risk factors, Artificial intelligence, Mental health, Risk assessment, Psychiatry
What's Your Reaction?
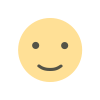
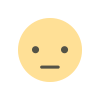
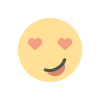
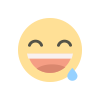
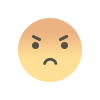
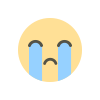
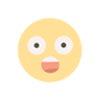