AI-Powered 3D Reconstruction Enhances Lung Surgery Planning
In a groundbreaking advancement set to transform thoracic surgery, researchers have harnessed the power of artificial intelligence to create unparalleled three-dimensional reconstructions of lung anatomy, significantly enhancing preoperative planning and patient outcomes. This pioneering effort, conducted by Chen, Dai, Peng, and colleagues, leverages cutting-edge machine learning techniques to faithfully model the complex spatial architecture of […]

In a groundbreaking advancement set to transform thoracic surgery, researchers have harnessed the power of artificial intelligence to create unparalleled three-dimensional reconstructions of lung anatomy, significantly enhancing preoperative planning and patient outcomes. This pioneering effort, conducted by Chen, Dai, Peng, and colleagues, leverages cutting-edge machine learning techniques to faithfully model the complex spatial architecture of the human lung, creating accurate, high-resolution 3D representations that provide surgeons with unprecedented insight prior to intervention. Published in Nature Communications, this work embodies a crucial leap forward in integrating AI with surgical science, promising to reshape how lung surgeries are approached and executed.
Lung surgery has long posed unique challenges due to the intricate configuration of bronchi, blood vessels, and parenchymal tissue. Traditional imaging modalities such as computed tomography (CT) scans offer only two-dimensional slices that surgeons must mentally reconstruct into three-dimensional form—a process prone to error and variability. The crux of this research centers on overcoming these limitations by deploying advanced deep learning models designed specifically to process and synthesize volumetric imaging data into volumetric, lifelike 3D maps of the lungs. These 3D visualizations enable clinicians to explore patient-specific anatomy dynamically and precisely, allowing for meticulous surgical planning that takes into account individual anatomical variations.
At the core of the approach is a novel AI framework that integrates convolutional neural networks with generative modeling to parse complex imaging data and reconstruct comprehensive lung structures, including distal alveolar regions, vascular trees, and bronchial networks. The model was trained on extensive datasets comprising thousands of annotated CT scans from diverse patient populations, ensuring robustness against anatomical variability. By learning subtle textural and morphological patterns, the AI not only delineates typical pulmonary structures but is also capable of detecting pathological deviations with remarkable sensitivity. This dual capability means surgeons can pinpoint tumor boundaries, assess vascular involvement, and better understand the spatial relationship of diseased tissue to vital anatomical landmarks.
The implications for lung cancer surgery, in particular, are profound. Precise delineation of tumor margins and adjacent tissues is vital for successful resections that maximize removal of malignant cells while preserving healthy lung function. The AI-enhanced 3D reconstructions facilitate preoperative virtual simulations where surgeons can plan optimal incision sites, resection extents, and reconstructive strategies. The dynamic nature of the models allows for interactive exploration at various angles, zoom levels, and even simulates tissue deformation, which closely mirrors real-life surgical scenarios. As a result, surgical teams can anticipate anatomical challenges and customize approaches tailored to each patient’s unique physiology and pathology.
Moreover, the system’s ability to integrate multimodal imaging inputs, including positron emission tomography (PET) along with CT data, enriches the informational content of the models by overlaying metabolic activity profiles on anatomical maps. This innovative data fusion helps distinguish aggressive tumor regions from benign tissue, aids in staging cancer accurately, and guides biopsy targeting. When combined with AI’s pattern recognition prowess, these comprehensive datasets elevate the precision of lung surgery planning beyond what has previously been achievable.
Technically, the researchers tackled significant hurdles involving image segmentation and reconstruction accuracy. They developed a multi-stage processing pipeline commencing with automated segmentation of lung components, followed by meshing algorithms that produce smooth, yet anatomically faithful, 3D surfaces. To enhance fidelity, a refinement step employing adversarial training was introduced, where generative adversarial networks (GANs) produced more realistic reconstructions by minimizing discrepancies between AI-generated models and real anatomical specimens. This adversarial approach mitigates noise and artifacts commonly seen in traditional reconstructions, resulting in clinically exploitable models.
Importantly, the AI system also incorporates uncertainty quantification mechanisms that highlight areas within the reconstruction where confidence is low due to imaging limitations or algorithmic ambiguity. These confidence maps serve as valuable guides for clinicians, delimiting regions that may require additional diagnostic attention or intraoperative verification. The incorporation of explainable AI principles ensures that the system’s outputs are interpretable and trustworthy, addressing a common concern surrounding black-box AI models in critical medical contexts.
The clinical validation phase demonstrated that surgeons using AI-driven 3D reconstructions achieved shorter operative durations, reduced intraoperative complications, and better preserved pulmonary function post-surgery compared to conventional planning methods. Surgeons reported heightened confidence and less cognitive fatigue when interacting with AI-generated models due to their clarity and comprehensiveness. These findings were further corroborated by radiologists and interdisciplinary teams who confirmed the anatomical accuracy and clinical relevance of the reconstructions across a variety of lung pathologies.
Beyond surgical planning, this technology holds promise for educational purposes, offering medical trainees immersive and interactive 3D lung models that improve their understanding of pulmonary anatomy and pathology. It also provides a platform for personalized patient education, allowing patients to visualize their own lung condition and the surgical plan, thereby enhancing informed consent and reducing anxiety related to the procedure.
Looking ahead, the research team envisions expanding the AI framework to accommodate real-time intraoperative updates by integrating data from surgical navigation systems and endoscopic imaging. This could enable surgeons to dynamically track anatomical changes during procedures and adapt plans instantly, ushering in an era of fully AI-augmented thoracic surgery. Furthermore, adaptations of this technology to other organ systems could catalyze a broad shift toward AI-assisted surgery across multiple specialties.
Integral to the translational success was the interdisciplinary collaboration among AI researchers, thoracic surgeons, radiologists, and biomedical engineers. Their combined expertise ensured the algorithms were clinically grounded, technically robust, and user-friendly. The open-access release of their code and datasets fosters ongoing innovation by the broader scientific community, accelerating the refinement and adoption of AI-driven 3D reconstructions in healthcare.
Challenges remain to be addressed, including ensuring the generalizability of AI models across diverse imaging devices and institutions, integrating complex multi-organ interactions, and complying with rigorous regulatory standards for clinical deployment. Nonetheless, this work marks a pivotal step in realizing AI’s promise to revolutionize precision surgery through enhanced anatomical visualization and decision support.
In sum, the research led by Chen and colleagues presents a transformative convergence of AI and surgical science. By delivering exquisitely detailed, patient-specific 3D lung reconstructions, their approach empowers surgeons with actionable insights that improve surgical accuracy and patient safety. This fusion of artificial intelligence with clinical practice exemplifies the future of medicine—where digital innovation meets human expertise to achieve unprecedented precision and personalization.
The implications of this research extend far beyond lung surgery. They suggest a paradigm shift in how complex anatomical information is processed and utilized across medicine, with AI serving as a powerful partner in unraveling biological complexity. As these technologies mature and permeate clinical workflows, patients stand to gain from procedures that are safer, more efficient, and tailored to their unique physiological makeup. The integration of AI-driven 3D reconstruction heralds a new chapter in surgical planning and intervention, one defined by clarity, confidence, and exceptional care.
—
Subject of Research: Artificial intelligence applications in medical imaging and surgical planning for lung surgery
Article Title: Artificial intelligence driven 3D reconstruction for enhanced lung surgery planning
Article References:
Chen, X., Dai, C., Peng, M. et al. Artificial intelligence driven 3D reconstruction for enhanced lung surgery planning.
Nat Commun 16, 4086 (2025). https://doi.org/10.1038/s41467-025-59200-8
Image Credits: AI Generated
Tags: 3D reconstruction for thoracic surgeryaccurate 3D lung modelsadvanced surgical planning techniquesAI in lung surgeryartificial intelligence in healthcaredeep learning models in medicineenhancing patient outcomes in surgerymachine learning in medical imagingNature Communications lung researchpreoperative planning with AItransforming surgical practices with AIvolumetric imaging for lung anatomy
What's Your Reaction?
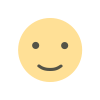
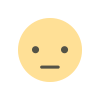
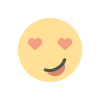
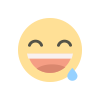
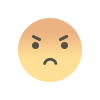
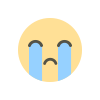
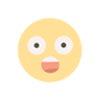