AI Model Forecasts Multi-Resistance Patterns in Bacteria
An innovative study spearheaded by researchers at Chalmers University of Technology and the University of Gothenburg has unveiled the significant capabilities of artificial intelligence (AI) in predicting the emergence of antibiotic resistance in bacteria. This study highlights the complexities of genetic data and how bacterial gene transfers can lead to the development of antibiotic-resistant strains. […]

An innovative study spearheaded by researchers at Chalmers University of Technology and the University of Gothenburg has unveiled the significant capabilities of artificial intelligence (AI) in predicting the emergence of antibiotic resistance in bacteria. This study highlights the complexities of genetic data and how bacterial gene transfers can lead to the development of antibiotic-resistant strains. The findings are particularly pertinent given the increasing global threat posed by antibiotic resistance, which poses severe challenges to public health.
Antibiotic resistance constitutes one of the most pressing health concerns, as stated by the World Health Organization (WHO). Infections caused by antibiotic-resistant bacteria represent a significant risk, complicating the treatment of diseases like pneumonia and sepsis. Often, patients undergoing surgical procedures or cancer treatments are at increased risk due to the presence of these resistant strains. The ability of bacteria to exchange genetic material underlies the rapid evolution of resistance, which underscores the need for comprehensive and data-driven approaches to combat this public health crisis.
The research team, led by Erik Kristiansson, a professor at Chalmers, utilized advanced AI models to examine historical gene transfer instances among various bacterial populations. By leveraging a dataset that encapsulates the DNA sequences of nearly one million bacteria, these investigators were able to provide a clearer picture of the dynamics driving antibiotic resistance. This extensive dataset is a culmination of collaborative efforts from the international scientific community over several years, emphasizing the power of shared knowledge in tackling complex health issues.
Focusing on the environments conducive to the gene transfer processes, the study unveiled key insights into the factors influencing the likelihood of antibiotic resistance development. Notably, it was found that environments such as wastewater treatment facilities and the human body serve as hotbeds for the exchange of resistance genes. Due to the elevated presence of antibiotics in these areas, bacteria carrying resistance traits are likely to encounter and share genes more frequently. Such environments thus play a pivotal role in the spread of resistance.
An essential aspect of this research is the genetic similarity between bacterial strains. The findings suggest that closely related bacteria are more inclined to share resistance genes, highlighting a critical evolutionary mechanism. This phenomenon arises because the energy costs of accepting foreign DNA are considerably lower among genetically similar species. The study suggests that understanding these dynamics can lead to better predictions of when antibiotic resistance is likely to emerge, thus aiding in the development of targeted strategies to mitigate its spread.
AI’s role in this research was instrumental, as it facilitated the analysis of complex biological interactions that are often difficult to quantify. The researchers engaged a robust AI model trained extensively on diverse datasets, which enabled them to explore the intricate relationships between genetic compatibility and gene transfer. It effectively illustrated how AI can transform large-scale biological data into actionable insights, influencing future research and public health strategies.
The team rigorously tested the model’s predictions against known instances of gene transfers and found it could accurately foresee these occurrences in a significant majority of cases. This validation step strengthens the model’s credibility as a diagnostic tool for identifying potential gene transfers in real-time, paving the way for preemptive actions to control the spread of resistance. The researchers emphasize that refining this model and expanding the dataset will enhance its predictive power.
Moving forward, the researchers envision the development of practical applications for the AI model, extending beyond theoretical implications. For instance, the model could be integrated into molecular diagnostic systems to detect emerging antibiotic-resistant strains in clinical settings or environmental monitoring systems for wastewater treatment plants. This would enable health authorities to implement timely interventions, minimizing the risk of outbreaks from resistant bacterial strains.
The implications of this study resonate far beyond academic circles. With antibiotic resistance leading to significant morbidity, mortality, and healthcare costs globally, the need for timely and effective interventions has never been more urgent. The ability to anticipate the emergence and spread of resistance genes could dramatically shift how public health systems respond to bacterial infections.
In conclusion, the innovative intersection of artificial intelligence and microbiology as demonstrated in this research represents a crucial advance in our understanding of antibiotic resistance. As researchers continue to harness these technologies, the potential to influence public health policy and improve clinical outcomes became more tangible. A collaborative approach leveraging AI can motivate researchers to keep probing the vast complexities of bacterial genetics, paving the way for groundbreaking treatments.
This game-changing research reflects a broader trend in utilizing data-driven methodologies to tackle longstanding issues in medicine. As the global health landscape evolves, it is essential to adapt and innovate, particularly in response to pressing challenges such as antibiotic resistance. Integrating AI within microbiology must be a priority for researchers aiming to improve patient outcomes and enhance public health systems throughout the world.
The urgency to act against the backdrop of antibiotic resistance can no longer be understated. With the tools and insights provided by advances in artificial intelligence, there is hope for not just managing but potentially reversing the alarming trends in antibiotic resistance. This collaborative effort of scientists worldwide underscores that global health challenges require global solutions, and AI might just be the ally we need to forge a path toward a healthier future.
Subject of Research: The study focuses on predicting antibiotic resistance through historical gene transfers in bacteria using artificial intelligence.
Article Title: Genetic compatibility and ecological connectivity drive the dissemination of antibiotic resistance genes
News Publication Date: 16-Mar-2025
Web References: DOI Link
References: Nature Communications
Image Credits: Chalmers University of Technology
Keywords: Artificial intelligence, antibiotic resistance, gene transfer, public health, machine learning, bacterial infections
Tags: advanced AI models in microbiologyAI in predicting antibiotic resistanceantibiotic-resistant strain developmentbacterial gene transfer mechanismsChalmers University of Technology researchcomprehensive approaches to combat antibiotic resistancedata-driven strategies in public health.genetic data analysis in bacteriapneumonia and sepsis treatment challengespublic health implications of antibiotic resistancesurgical patients and antibiotic resistance risksWorld Health Organization antibiotic resistance concerns
What's Your Reaction?
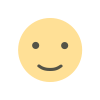
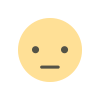
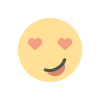
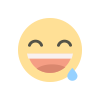
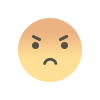
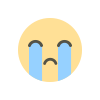
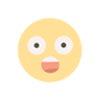