AI Delivers Dependable Answers While Reducing Computational Demands
In the rapidly evolving landscape of artificial intelligence, researchers have long grappled with the dual-edged sword of precision and uncertainty presented by large language models (LLMs). These powerful AI engines possess the ability to produce answers with remarkable accuracy, yet they simultaneously have the capacity to generate responses that range from insightful to nonsensical. This […]

In the rapidly evolving landscape of artificial intelligence, researchers have long grappled with the dual-edged sword of precision and uncertainty presented by large language models (LLMs). These powerful AI engines possess the ability to produce answers with remarkable accuracy, yet they simultaneously have the capacity to generate responses that range from insightful to nonsensical. This inconsistency can pose significant challenges, particularly when determining the reliability of information derived from such models. The complexity lies in the manner in which LLMs interpret and manage uncertainty within their responses.
A dedicated team at the Institute for Machine Learning within the Department of Computer Science at ETH Zurich has unveiled an innovative approach aimed at mitigating uncertainty in AI outputs. This breakthrough method, known as the SIFT algorithm—short for Selecting Informative Data for Fine-Tuning—enables the integration of specialized data directly into general language models. By enhancing the foundational knowledge of these models with subject-specific information, this algorithm significantly improves the quality of responses generated in response to complex queries.
The implications of SIFT’s capabilities are particularly profound for professionals in specialized fields such as scientific research or corporate industries where a deeper, more nuanced understanding is imperative. The algorithm allows users to input data that may not be universally available to the general training datasets of LLMs. As articulated by the lead developer, Jonas Hübotter, this enhancement enables AI to not only access vast amounts of general knowledge but also to delve into contexts that require detailed, domain-specific insights.
SIFT operates by utilizing the intricate relationships that exist within the language data mapped out in the multidimensional space of the AI’s architecture. The way information is organized within LLMs can be visualized through a network of vectors, which define the semantic and syntactic relationships among various data points. As these models are trained, they develop vectors that capture their relationships, allowing for a more refined understanding of the nuances that distinguish one piece of data from another.
An integral feature of SIFT lies in its capability to evaluate correlations based on vector angles, illustrating how closely related pieces of information are to one another. When two vectors align closely, they indicate a strong relevance to the core inquiry posed by the user. Through this mechanism, SIFT can intelligently discern which pieces of information complement each other, thus optimizing the response process. The equation underlying this methodology directly correlates the angles of these vectors to the relevance of their associated content, allowing for a focused extraction of pertinent data.
In stark contrast to traditional methods, such as the nearest neighbor approach, which tends to accumulate redundant information, SIFT prioritizes diversity in perspectives. For instance, consider a query that encompasses multiple aspects of a subject. Using the nearest neighbor method, AI might deliver overlapping responses that relate to varying facets of a single issue, such as a person’s age without addressing their family or career details adequately. In this example, while LLMs may regurgitate information about Roger Federer’s age, they could neglect equally vital information about his children simply because it exists in a less frequent context within the training data.
The SIFT algorithm also enhances computational efficiency in AI applications by employing a strategy termed test-time training. This innovation allows the model to adaptively assess how much data is necessary to yield reliable responses. By consistently refining the selection criteria based on user query specifics, SIFT facilitates an efficient allocation of computational resources, ensuring that the AI can produce high-quality responses without unnecessarily taxing computational power.
Notably, in trials utilizing standardized datasets, the models augmented with SIFT surpassed the performance of some of the most advanced current AI systems, achieving comparable efficacy while operating at nearly one-fortieth of the model size. This finding underscores the potential of SIFT not only to enhance the precision of AI responses but also to democratize sophisticated AI tools, making them accessible to a broader range of applicants without the prohibitive resource demands typically associated with large-scale models.
Moreover, the capacity of SIFT to detail the specific enrichment data selected for a given prompt opens up new avenues for application beyond traditional text-based queries. For instance, in medical diagnostics, the algorithm could assist practitioners in identifying which laboratory results or test measurements are most relevant for a particular diagnosis. This aligns with contemporary advances in personalized medicine, where tailored insights can significantly impact patient outcomes.
The introduction of the SIFT algorithm marks a significant milestone in the ongoing dialogue around AI in academia and industry. By addressing one of the foremost challenges in AI—uncertainty—the ETH Zurich researchers contribute not only to theoretical advancements in our understanding of machine learning but also practical solutions that promise to revolutionize the way we interact with these transformative technologies. Their forthcoming presentation of this work at the International Conference on Learning Representations in Singapore is set to further spotlight the algorithm’s impact on refining AI capabilities.
As the discourse surrounding AI continues to evolve, validation of methodologies such as SIFT will play an increasingly critical role. By implementing techniques aimed at reducing uncertainty and enhancing specificity, researchers can forge pathways toward AI systems that are not only more reliable but also more attuned to the complexities of human inquiry. As we stand on the brink of new advancements in the field, it remains essential to explore how enriched AI can transform our collective capabilities.
In conclusion, the ongoing work at ETH Zurich clearly emphasizes the importance of reducing uncertainty within AI systems. The SIFT algorithm is a testament to how nuanced and coherent responses can be shaped by fostering a deeper understanding of the relationships between various data points. This represents a substantial leap forward in the quest for trustworthy, effective AI solutions that can adapt to the complexities of real-world applications.
Subject of Research:
Article Title:
News Publication Date:
Web References:
References:
Image Credits:
Keywords
Tags: advancements in artificial intelligence technologyAI language modelsartificial intelligence in scientific researchcomplex query handling in AIenhancing AI reliabilityETH Zurich machine learning researchimproving AI response accuracymitigating uncertainty in AIprecision vs uncertainty in AISIFT algorithm for fine-tuningspecialized data integration in AItrustworthiness of AI-generated information
What's Your Reaction?
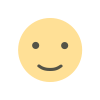
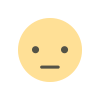
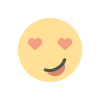
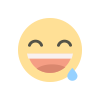
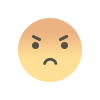
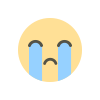
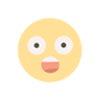