AI: A Game Changer in Choosing the Right Doctor
In an era dominated by technological advances, the healthcare domain finds itself at a pivotal juncture, particularly in terms of how patients select their medical providers. Maytal Saar-Tsechansky, a prominent professor of information, risk, and operations management at the Texas McCombs School of Business, has identified a critical gap in the decision-making processes of patients […]

In an era dominated by technological advances, the healthcare domain finds itself at a pivotal juncture, particularly in terms of how patients select their medical providers. Maytal Saar-Tsechansky, a prominent professor of information, risk, and operations management at the Texas McCombs School of Business, has identified a critical gap in the decision-making processes of patients when it comes to choosing doctors. Relying on personal recommendations, physician personalities, or seemingly superficial factors like the decor of an office, patients find themselves lacking crucial information on a doctor’s diagnostic accuracy or their overall track record.
The importance of credible and comprehensive information has never been more apparent, especially as artificial intelligence becomes an integral part of the medical landscape. With systems increasingly employing AI for diagnostic support, the challenge arises: how do we evaluate the effectiveness of human practitioners when augmented by sophisticated algorithms? Here, Saar-Tsechansky posits the need for a framework that not only evaluates doctors but also allows patients to understand the implications of their choices better.
To tackle this pressing issue, Saar-Tsechansky, alongside her doctoral student Wanxue Dong and Tomer Geva from Tel Aviv University, developed a groundbreaking machine learning framework termed MDE-HYB. This innovative algorithm integrates two distinct yet complementary streams of information: one that compiles historical data reflecting the quality of an expert’s past decisions and another that performs a granular assessment of specific cases. This dual approach enables a more nuanced evaluation of expertise within a range of professions, spanning from medical practitioners to engineers.
The researchers’ comparisons between MDE-HYB and other evaluators revealed impressive results. The benchmark included three alternative algorithms as well as assessments from 40 human evaluators. Observing MDE-HYB’s performance across disparate data sets—sales tax audits, spam detection, and online movie reviews—it became clear that the algorithm consistently outperformed its competitors. Remarkably, MDE-HYB boasted error rates that were up to 95% lower than its algorithm counterparts and up to 72% lower when set against human evaluators. These numbers signify an impressive leap in evaluation accuracy within decision-making processes.
In a thorough examination of Saar-Tsechansky’s original concern about medical diagnostics, MDE-HYB specifically analyzed the historical accuracy of doctors. For instance, it demonstrated a 41% reduction in average misdiagnosis rates when compared to selections made through alternative algorithms—an outcome that could have far-reaching implications for patient care. If effectively implemented, this reduction in misdiagnoses could translate to significant improvements in health outcomes, which would inevitably lead to reduced healthcare costs.
While the findings are promising, Saar-Tsechansky emphasizes that MDE-HYB currently requires further refinement before it can be practically applied in real-world scenarios. Through her research paper, she aims to ignite discussion among scholars and practitioners alike, encouraging others to expand upon and enhance this innovative method. The potential applications of such a framework are vast, extending beyond healthcare into any profession involving critical decision-making, such as finance, engineering, and legal services.
The implications of Saar-Tsechansky’s work are profound. As regulators and managers grapple with the necessity to monitor expert decision-making, MDE-HYB presents a feasible solution. Its adoption could lead to timely interventions for professionals in need of improvement, ultimately increasing accountability within various fields. This could serve not only to elevate professional standards but also empower consumers, enabling them to make informed choices about service providers like doctors.
Every profession entails responsibility, particularly when decisions carry significant consequences. The demand for transparency and accountability in decision-making cannot be overstated, and innovation in this arena will ensure that professionals remain accountable for their judgments. MDE-HYB stands as a testament to the positive disruptions that artificial intelligence can introduce within sectors traditionally dominated by human intuition and experience.
In summary, the importance of this research lies not only in its technical accomplishments but also in its potential impact on society as a whole. By harnessing machine learning to scrutinize and enhance expert decision-making, we stand at the threshold of a new era where informed choices become the norm. Enhanced decision quality within fields requiring expert judgment can lead to improved outcomes for individuals and organizations alike. This work by Saar-Tsechansky and her associates represents a vital step forward in a globally evolving landscape of healthcare and beyond.
As investigations like these progress, they underscore the necessity for continual advancements in both the sciences and ethical frameworks that guide expert evaluations. In a world increasingly characterized by complexity and uncertainty, having reliable systems in place to assess those who make decisions that affect public welfare is not just beneficial; it is imperative.
In conclusion, the future of decision-making in critical professions promises to be smarter and more accountable, propelled by innovative research. With MDE-HYB as a stepping stone, the next chapter in evaluating expertise could reshape our understanding and engagement with those who wield significant influence over the lives of others.
Subject of Research: Machine Learning Framework for Assessing Experts’ Decision Quality
Article Title: A Machine Learning Framework for Assessing Experts’ Decision Quality
News Publication Date: 15-Oct-2024
Web References: Management Science
References: Relevant research and articles pertaining to AI in healthcare and expert evaluation.
Image Credits: None specified.
Keywords: Machine Learning, Decision-Making, Artificial Intelligence, Healthcare, Expert Evaluation, MDE-HYB, Patient Care.
What's Your Reaction?
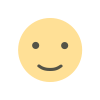
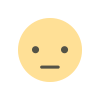
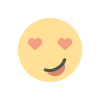
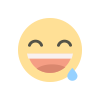
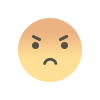
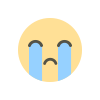
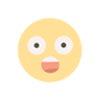