Advancing Toward Complete Automation in English Proficiency Assessment
In the digital age, the pursuit of effective language learning strategies has become paramount, not only for personal development but also for professional and educational advancement. One particularly captivating method that has emerged is called elicited imitation (EI), where learners listen to sentences and repeat them back with precision. This technique offers deep insights into […]

In the digital age, the pursuit of effective language learning strategies has become paramount, not only for personal development but also for professional and educational advancement. One particularly captivating method that has emerged is called elicited imitation (EI), where learners listen to sentences and repeat them back with precision. This technique offers deep insights into a learner’s command of a language, engaging not just their memory but their overall linguistic competence. The necessity of having a profound understanding of language patterns and structures becomes increasingly critical, especially when sentences extend beyond the confines of working memory, commonly ranging from 8 to 10 syllables. Here, EI serves as a revealing lens on the true proficiency of language learners.
Despite its beneficial attributes, the widespread application of EI has been hindered by some significant challenges, particularly the requirement for trained human evaluators to assess each response. This painstaking measure not only consumes substantial time but also limits the scalability of assessments that could otherwise benefit a larger group of learners. Even with a standardized scoring system developed in the early 2000s, EI’s resource demands remain a barrier in educational frameworks where such assessments could yield significant benefits. This limitation has prompted scholars to seek innovative solutions to leverage technological advancements in language assessment.
Amidst this backdrop of challenges, researchers have taken a transformative step forward. A team led by Associate Professor Michael McGuire from the Department of English at Doshisha University in Japan, alongside Dr. Jenifer Larson-Hall from The University of Kitakyushu, has initiated groundbreaking research into automating EI assessment. Their recent publication, made available online on March 11, 2025, and featured in Volume 4, Issue 1 of Research Methods in Applied Linguistics shortly after, unveils the potent capabilities of artificial intelligence in revolutionizing how language proficiency is measured.
The duo’s research posits an ingenious two-part methodology designed to automate EI testing. The first facet utilizes OpenAI’s Whisper, an advanced automatic speech recognition (ASR) system renowned for its adaptability and accuracy, particularly with non-native speakers and in noisy environments, to transcribe learners’ spoken answers into text. This technology not only allows for rapid processing but also ensures high fidelity in capturing linguistic nuances. Following transcription, the second element employs a computational metric known as Word Error Rate (WER), which assesses the degree of accuracy of spoken responses against the original prompts, meticulously identifying any discrepancies such as substitutions, insertions, and deletions at the word level.
The hypothesis behind integrating these technologies is profound; they could feasibly replace human raters while delivering consistent assessment quality. The research team conducted their evaluation with 30 Japanese university students who were presented with a 30-item EI test, procuring an impressive 900 speech samples that were evaluated through both human raters and the Whisper ASR system. The findings were astonishing: the transcriptions from Whisper bore remarkable similarity to those generated by human evaluators, underscoring the system’s reliability and robustness—even under less-than-ideal testing conditions, including various background noises, an aspect that is often prevalent in real-world educational settings.
The significance of the automated scoring process was underscored by its remarkable alignment with traditional human evaluations, showcasing a correlation that approached perfection. This facet of the study is vital as it not only validates the reliability of employing AI for language proficiency assessments but also opens the door to applications that could streamline testing processes and make them more accessible. As Mr. McGuire articulates, their study affirms the viability and trustworthiness of fully automated speaking evaluations, which can easily be scaled up at a lower cost—crucial for institutions that encounter budget constraints.
The shift towards automation in language assessment holds more than just the promise of saving educators time and resources; it paves the way for more frequent evaluations of students’ speaking abilities. Such regular feedback is indispensable for language development, allowing educators and learners alike to track progress in real time. Additionally, this automated approach facilitates the conduction of larger-scale studies, which were previously hampered by the exhaustive nature of manual scoring, offering a fresh avenue for research and development in educational linguistics.
Looking ahead, the research team continues to forge a path in the realm of automated language assessment. They are currently developing a web-based EI testing platform that will utilize both Whisper ASR and WER, allowing learners to undertake tests conveniently on smartphones with scoring that occurs online in real-time. This endeavor aims to make access to automated language assessments available not only to researchers but also to educators who seek modern solutions for evaluating spoken language proficiency.
The future aspirations of McGuire’s research team extend further into enhancing the breadth and efficacy of language assessments. They envision creating multiple standardized test forms characterized by equivalent difficulty levels, providing a reliable means of tracking language development over time. Furthermore, adapting these assessments to align with specific curricula—focusing on targeted vocabulary and grammar—could facilitate a more personalized learning experience. The ultimate goal also includes the potential development of adaptive tests that pinpoint oral proficiency with an efficiency that surpasses traditionally established methods.
In a world where artificial intelligence is increasingly embedded in various aspects of education and daily life, this pioneering research exemplifies a significant leap toward making reliable language assessment both scalable and accessible. As the landscape of language learning continues to evolve, the integration of advanced technologies such as Whisper ASR in automated assessments will undoubtedly reshape educational paradigms, delivering innovative solutions that align with the needs of learners and educators alike.
In conclusion, the convergence of elicited imitation techniques with cutting-edge artificial intelligence not only addresses existing challenges in language assessment but also heralds a new era in educational methodology. By embracing these innovations, the academic community stands to gain invaluable resources in the pursuit of effective language education—resources that can ultimately empower learners to achieve language proficiency with greater confidence and accuracy.
Subject of Research: Language Proficiency Assessment
Article Title: Assessing Whisper automatic speech recognition and WER scoring for elicited imitation: Steps toward automation
News Publication Date: 1-Apr-2025
Web References: http://dx.doi.org/10.1016/j.rmal.2025.100197
References: None cited
Image Credits: Michael McGuire from Doshisha University, Japan
Keywords
Automatic Speech Recognition, Language Assessment, Elicited Imitation, Word Error Rate, Artificial Intelligence, Education Technology, Language Learning, Proficiency Measurement, Scalable Testing, Innovation in Education
Tags: advancements in language assessment technologyautomated language evaluationchallenges in language assessmentdigital language learning strategieselicited imitation techniquehuman evaluators in educationlanguage proficiency assessmentlinguistic competence measurementmemory and language learningprofessional development through language learningscalability of language testsstandardized scoring systems in education
What's Your Reaction?
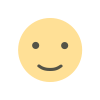
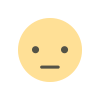
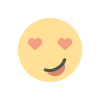
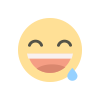
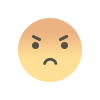
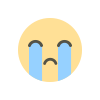
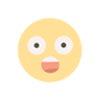