Advanced Photoacoustic Microscopy: Integrating Physics and Deep Learning for Improved Imaging
In the realm of medical imaging, photoacoustic microscopy (PAM) stands at the forefront of innovation, marrying the principles of optics and acoustics to provide unprecedented insights into tissue and cellular structures. Recent advancements introduced the concept of physics-embedded degradation learning (PEDL), a novel methodology that aims to enhance the capabilities of PAM. This method integrates […]

In the realm of medical imaging, photoacoustic microscopy (PAM) stands at the forefront of innovation, marrying the principles of optics and acoustics to provide unprecedented insights into tissue and cellular structures. Recent advancements introduced the concept of physics-embedded degradation learning (PEDL), a novel methodology that aims to enhance the capabilities of PAM. This method integrates deep learning with fundamental physical principles, presenting an exciting avenue for improving image quality and diagnostic potential.
Photoacoustic imaging (PAI) works by utilizing laser light to illuminate a target, typically biological tissue. When the laser energy is absorbed, it induces a local temperature rise, resulting in pressure fluctuations that generate sound waves. These sound waves are then captured and translated into images that reveal the internal anatomy and functional conditions of the target. High-resolution imaging coupled with a significant penetration depth positions PAI as a compelling technique, particularly in medical contexts.
PAM, an advanced form of PAI, offers an enhanced spatial resolution, enabling researchers to examine tissues and cellular structures at a microscopic level. The precision of PAM is especially vital in biomedical research, where understanding subcellular details can guide therapeutic approaches and disease diagnostics. Nevertheless, PAM faces ongoing challenges, including noise interference and signal attenuation, particularly during deep-tissue imaging.
To tackle these difficulties, researchers have turned their focus toward integrating advanced technologies such as high-performance detectors and sophisticated algorithms informed by deep learning methodologies. However, conventional PAM still grapples with limitations concerning accuracy, particularly amid varying experimental conditions and complex biological environments. The quest for substantial improvements in PAM’s overall performance remains critically important for its application in clinical settings.
Professors Qian Chen and Chao Zuo from Nanjing University of Science and Technology have made significant strides in addressing these technological hurdles. Their innovative PEDL method seamlessly integrates the physical laws governing PAM with a comprehensive deep learning framework. By leveraging the intrinsic optical and acoustic properties of tissues, PEDL is designed to mimic degradation processes, resulting in a more accurate representation of the conditions affecting PAM imaging.
The structural backbone of the PEDL framework is based on the U-Net architecture, renowned for its effectiveness in image segmentation tasks. Featuring multiple residual blocks and a Global Context (GC) attention module, PEDL exhibits a unique ability to extract complex features from PAM images. The incorporation of residual blocks not only enhances feature extraction but also mitigates common pitfalls such as the vanishing gradient problem, which can hinder the training of deep neural networks.
Moreover, the GC self-attention mechanism enriches the model’s capability by facilitating the understanding of contextual information throughout the feature map. This is particularly essential for capitalizing on the nuances present in PAM images, which can include minute variations in structure due to noise or other interferences. By enhancing the contextual awareness of the network, PEDL empowers researchers to make more informed predictions, particularly in challenging imaging scenarios.
Results from employing the PEDL method have demonstrated its profound impact on image reconstruction processes within PAM. For instance, experiments have shown a marked improvement in image clarity post-reconstruction, even in cases characterized by severe degradation and noise interference. The enhancements in resolution achieved through this reconstruction process are particularly promising for visualizing complex biological structures, such as blood vessels, which can often be obscured by surrounding noise.
In practical application, the PEDL framework has shown to bolster the performance of PAM, particularly when faced with various challenges such as energy variation and noise fluctuations. By improving the capacity to discern fine structures within the imaged samples, researchers can potentially enhance the efficacy of PAM in diverse biomedical applications, paving the way for more reliable diagnostics and innovative therapeutic strategies.
As the realm of deep learning continues to evolve, integrating physical models into imaging technologies holds considerable promise. By combining the analytical prowess of deep neural networks with a robust understanding of physical principles, researchers are poised to elevate the quality of PAM imaging. This not only augurs well for advancing basic scientific research but also emphasizes the potential for translating these innovations into clinical practice.
In summary, the intersection of physics, computer science, and biomedical engineering represented by the PEDL initiative illustrates a significant leap forward in photoacoustic microscopy. The integration of deep learning paradigms with heavy reliance on foundational physical concepts embodies a holistic approach to overcoming existing limitations in the field. Moving forward, further investigations into leveraging physical insights within deep learning frameworks are likely to yield continued enhancements in imaging technologies, ultimately benefiting medical diagnostics and treatment outcomes.
In conclusion, as photoacoustic microscopy continues to mature as a technology, its reliance on innovations like PEDL will be crucial in unlocking deeper insights into biological processes. This emerging amalgamation of disciplines is set to redefine what is possible within live imaging applications, establishing a new standard for quality and reliability that could revolutionize the landscape of biomedical research and clinical diagnostics.
Subject of Research: Enhanced photoacoustic microscopy with physics-embedded degeneration learning
Article Title: Enhanced Photoacoustic Microscopy: Leveraging Deep Learning and Physical Principles
News Publication Date: [Insert Date]
Web References: [Insert Links]
References: [Insert References]
Image Credits: OEA
Keywords
photoacoustic microscopy, deep learning, high quality imaging, physical model
Tags: advanced photoacoustic microscopybiomedical research imagingdeep learning in medical imagingdiagnostic potential of photoacoustic microscopyhigh-resolution imaging methodsinnovative imaging solutions in healthcarenoise reduction in imagingoptical and acoustic imaging integrationphotoacoustic imaging techniquesphysics-embedded degradation learningsignal attenuation in photoacoustic microscopytissue and cellular imaging
What's Your Reaction?
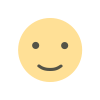
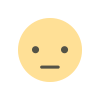
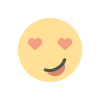
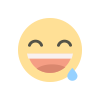
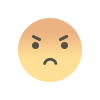
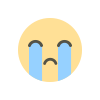
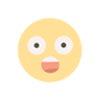