A biased edge enhancement method for truss-based community search
Community Search, whose target is to search for a cohesive and meaningful community containing the query node, has drawn intense research interest and had extensive applications in various real-world networks recently. In contrast to lower-order methods from individual nodes and edges, the truss-based method aims to explore the truss structure of complex networks. Nevertheless, the […]
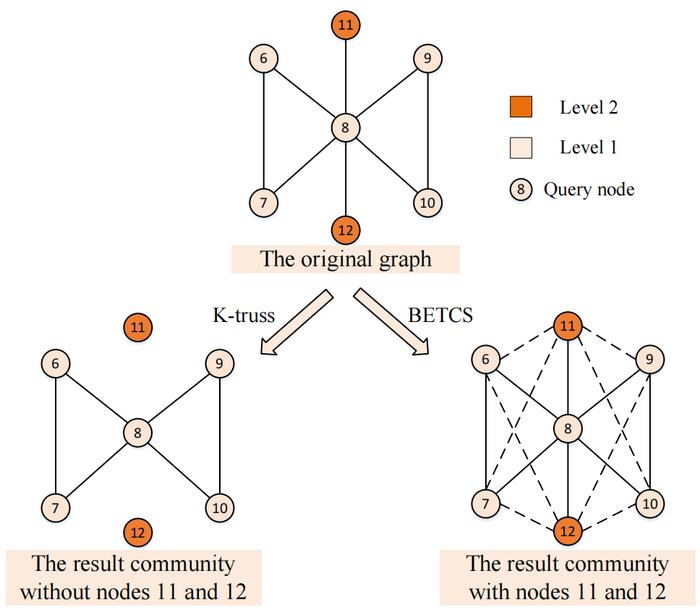
Community Search, whose target is to search for a cohesive and meaningful community containing the query node, has drawn intense research interest and had extensive applications in various real-world networks recently. In contrast to lower-order methods from individual nodes and edges, the truss-based method aims to explore the truss structure of complex networks. Nevertheless, the truss-based hypergraph constructed from the original connected graph is usually fragmented into numerous subgraphs and isolated nodes.
Credit: Yuqi LI, Tao MENG, Zhixiong HE, Haiyan LIU, Keqin LI
Community Search, whose target is to search for a cohesive and meaningful community containing the query node, has drawn intense research interest and had extensive applications in various real-world networks recently. In contrast to lower-order methods from individual nodes and edges, the truss-based method aims to explore the truss structure of complex networks. Nevertheless, the truss-based hypergraph constructed from the original connected graph is usually fragmented into numerous subgraphs and isolated nodes.
To solve the problems, a research team led by Tao Meng published their new research on 14 Mar 2024 in Frontiers of Computer Science co-published by Higher Education Press and Springer Nature.
The team proposed a biased edge enhancement method to preserve and enhance the higher-order connectivity in hypergraphs with the fragmentation issue. Extensive experiments are performed on various networks to show that our method can effectively address the fragmentation issue and performs better than some state-of-the-art methods.
In the research, they mainly study the community search problem based on higher-order structures. Community Search, whose target is to search for a cohesive and meaningful community containing the query node, has drawn intense research interest and had extensive applications in various real-world networks recently. In contrast to lower-order methods from individual nodes and edges, the truss-based method aims to explore the truss structure of complex networks. Nevertheless, the truss-based hypergraph constructed from the original connected graph is usually fragmented into numerous subgraphs and isolated nodes. To address the fragmentation issue faced by most of the existing truss-based community search methods, they propose a biased edge enhancement method to preserve and enhance the higher-order connectivity in hypergraphs with the fragmentation issue.
Initially, a coarse subgraph containing the desired communities is identified based on the query nodes. Then, measure the proximity of each node in the subgraph to it. Furthermore, these nodes are divided into several levels based on their proximity values, with nodes at lower levels having higher proximity values. The edges are then enhanced by connecting lower-level nodes to nodes at the level above them. Finally, an edge-enhanced k-truss community search is performed on the subgraph to obtain the desired communities. Extensive experiments on various networks show that the proposed method can effectively solve the fragmentation problem and perform better than some state-of-the-art methods.
DOI: 10.1007/s11704-024-2604-8
Journal
Frontiers of Computer Science
DOI
10.1007/s11704-024-2604-8
Method of Research
Experimental study
Subject of Research
Not applicable
Article Title
A biased edge enhancement method for truss-based community search
Article Publication Date
14-Mar-2024
What's Your Reaction?
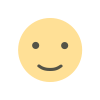
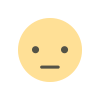
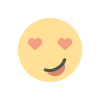
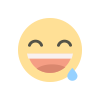
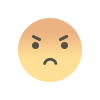
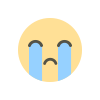
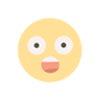